Voluntary Response Sampling: Pros, Cons & Examples
Voluntary response sampling, a non-probability sampling method, relies heavily on the active participation of individuals, who, unlike in probability sampling methods such as stratified sampling, self-select into the sample. This approach is frequently observed in customer feedback surveys, where SurveyMonkey tools are used to gather opinions from those willing to respond, potentially skewing the data. Critics, including those within the American Statistical Association, often point out that the resulting data might not accurately represent the broader population, introducing significant bias. Consider, for instance, call-in polls conducted by media outlets; the participants typically hold strong opinions, leading to skewed outcomes that do not reflect the views of a larger demographic.
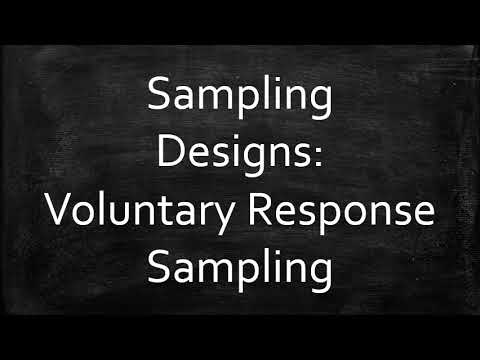
Image taken from the YouTube channel Stat Brat , from the video titled Sampling Designs: Voluntary Response Sampling .
The Unseen Challenge: Bias in Survey Research
Bias, the silent saboteur of accuracy, poses a formidable challenge across all domains that rely on survey research. From political polling to market analysis, and from public health studies to academic inquiries, the pervasive nature of bias threatens the integrity of our data-driven insights. Ignoring this challenge can lead to flawed conclusions, misguided strategies, and ultimately, poor decision-making.
Sound Statistical Methodology: The Foundation of Reliable Research
At the heart of sound survey research lies a commitment to fundamental statistical principles. These principles dictate that our samples must be representative of the populations they aim to describe.
Data collection methods must be rigorous and standardized to minimize measurement error. And data analysis must be transparent and objective, free from the influence of preconceived notions or vested interests.
When these principles are compromised, the resulting data becomes unreliable, and the conclusions drawn from it become suspect.
Sampling Bias: A Distortion of Reality
Among the various forms of bias, sampling bias stands out as a particularly insidious threat. It arises when the sample selected for a survey does not accurately reflect the characteristics of the population from which it is drawn.
This can occur in several ways, leading to systematic distortions in the results. We will be focusing on three main types of sampling bias: sampling bias itself, self-selection bias, and non-response bias.
Sampling bias can occur when a researcher uses a non-random sampling method, self-selection bias when individuals volunteer to participate, and non-response bias when certain groups are less likely to respond to a survey. Each of these biases introduces a systematic error that can skew the results and undermine the validity of the research.
Addressing these biases is crucial for ensuring the reliability and accuracy of survey research.
Unmasking Sampling Bias: A Trio of Threats
Having established the ubiquity of bias in survey research, it is now imperative to dissect its most insidious forms. Sampling bias, self-selection bias, and non-response bias constitute a triumvirate of threats that can systematically distort survey results, rendering them not merely inaccurate but dangerously misleading. A comprehensive understanding of these biases is the first crucial step towards mitigating their influence.
The Insidious Nature of Sampling Bias
Sampling bias arises when the sample selected for a survey is not representative of the population it purports to represent. This occurs when some members of the population are systematically more likely to be selected than others, leading to a skewed representation of the overall population.
Imagine, for instance, a survey on consumer preferences for automobiles conducted exclusively at luxury car dealerships. The results would undoubtedly be skewed towards high-end vehicles, failing to capture the preferences of the broader population, including those who purchase more affordable cars.
Another example is conducting a political poll only by calling landlines. This excludes a growing segment of the population who rely solely on mobile phones, potentially skewing the results towards older demographics and those with more traditional lifestyles.
These scenarios illustrate how sampling bias can introduce systematic errors, leading to inaccurate generalizations about the population of interest.
The Allure and Peril of Self-Selection Bias
Self-selection bias emerges when individuals choose whether or not to participate in a survey. This is particularly problematic because those who opt to participate are often systematically different from those who do not, leading to a non-representative sample.
Consider an online survey asking for feedback on a company's customer service. Individuals who had particularly positive or negative experiences are far more likely to respond than those with neutral opinions. This creates a skewed representation of overall customer satisfaction, as the voices of those with moderate experiences are underrepresented.
Another common example is online reviews. People who are extremely happy or angry are more likely to leave a review. Those who have an experience between these extremes might not feel as compelled to leave a review.
Similarly, voluntary participation in research studies can attract individuals with a particular interest in the topic, potentially biasing the results. The very act of choosing to participate introduces a layer of non-randomness, undermining the representativeness of the sample.
The Silent Distortion of Non-Response Bias
Non-response bias occurs when a significant proportion of individuals selected for a survey do not respond, and these non-respondents differ systematically from those who do respond. This can compromise the validity of the survey results, even if the initial sample was carefully selected.
Imagine a mail survey sent to a random sample of households. If the response rate is low, it is likely that those who did respond differ from those who did not.
For example, individuals with lower literacy levels or those who are less engaged with the topic of the survey may be less likely to respond.
This can lead to a distorted representation of the population, as the views and experiences of non-respondents are not captured.
Quantifying non-response bias is a challenging but crucial task. Researchers can attempt to compare the characteristics of respondents to known demographic data for the population. They may also conduct follow-up surveys with a small sample of non-respondents to assess how their views differ from those of the initial respondents.
Margin of Error: A False Sense of Security
The margin of error is often presented as the ultimate arbiter of survey accuracy, a seemingly straightforward metric that quantifies the uncertainty inherent in sampling a larger population. However, placing undue faith in this statistic, particularly in the presence of underlying biases, is akin to navigating a treacherous sea with a faulty compass. While the margin of error provides a quantifiable range around survey results, it offers a false sense of security if systematic biases are left unaddressed.
The Illusion of Precision
The margin of error, typically expressed as a plus or minus percentage, reflects the expected range within which the true population value is likely to fall. It is a function of sample size and the variability within the sample. A smaller margin of error suggests greater precision.
However, this precision is predicated on the assumption of a random and representative sample. When biases creep into the sampling process, this fundamental assumption is violated, rendering the margin of error a misleading indicator of accuracy.
Bias Amplification: When Error Magnifies
Bias, unlike random error, introduces a systematic distortion in the survey results, pushing them consistently in one direction.
This skew can significantly exacerbate the impact of the margin of error. Imagine a survey designed to gauge public support for a particular policy. If the survey is administered primarily to individuals who are already known to favor the policy, the results will be skewed towards higher support.
Even if the margin of error is small, the true level of support in the overall population could be significantly lower than what the survey indicates. The margin of error, in this case, merely reflects the precision within the biased sample, not the accuracy of the estimate for the entire population.
The Blind Spot of Unacknowledged Bias
Relying solely on the margin of error can create a blind spot, obscuring the presence and magnitude of underlying biases. Researchers might be lulled into a false sense of confidence, believing that their results are accurate simply because the margin of error is acceptably small.
This is especially dangerous in situations where the bias is subtle or difficult to detect. A seemingly well-designed survey could still be plagued by unacknowledged biases that significantly distort the findings.
Beyond the Margin: Accounting for the Unseen
The key to responsible survey research lies in acknowledging the limitations of the margin of error and actively seeking to identify and mitigate potential biases. This requires a multifaceted approach that extends beyond simply calculating the margin of error.
Strategies for Bias Mitigation
-
Careful Survey Design: The design of the survey itself is paramount. Questions should be clear, unbiased, and carefully worded to avoid leading respondents.
-
Diverse Sampling: Strive for a sample that is representative of the target population in terms of demographics, attitudes, and other relevant characteristics.
-
Weighting and Adjustment: Statistical techniques, such as weighting, can be used to adjust the sample data to better reflect the known characteristics of the population.
-
Transparency and Disclosure: Clearly articulate the limitations of the survey, including potential sources of bias and their possible impact on the results.
Redefining Accuracy: A Holistic Perspective
While the margin of error provides a valuable measure of sampling variability, it should not be considered the sole indicator of survey accuracy.
A truly accurate survey is one that not only minimizes random error but also actively addresses and mitigates potential biases. By adopting a holistic perspective and embracing a culture of transparency, researchers can move beyond the false sense of security offered by the margin of error and strive for a more nuanced and reliable understanding of the world around them.
Designing for Accuracy: Robust Survey Methodologies
The margin of error is often presented as the ultimate arbiter of survey accuracy, a seemingly straightforward metric that quantifies the uncertainty inherent in sampling a larger population. However, placing undue faith in this statistic, particularly in the presence of underlying biases, is akin to navigating treacherous waters with a faulty compass. A truly reliable survey hinges on meticulous design – a robust methodology capable of preemptively minimizing bias and maximizing the fidelity of the data collected.
The Cornerstones of Effective Survey Design
An effective survey design is not merely a collection of questions; it's a carefully constructed instrument calibrated to elicit accurate and representative responses. Several key elements contribute to its overall robustness.
First, clearly defined objectives are paramount. What specific insights are you seeking? What population are you targeting? Ambiguity at this stage can lead to unfocused questions and ultimately, skewed results.
Second, a well-structured questionnaire is crucial. This encompasses question order, response options, and overall flow. Leading questions, double-barreled questions (asking two things at once), and confusing language must be avoided.
Third, a carefully considered sampling strategy is essential. The goal is to obtain a sample that accurately reflects the characteristics of the target population. This might involve random sampling, stratified sampling, or other techniques tailored to the specific research objectives.
Question Construction: Avoiding the Bias Trap
The way a question is worded can profoundly influence the responses it elicits. Even subtle changes in phrasing can introduce bias, leading to inaccurate or misleading results.
Consider these examples:
-
Biased: "Wouldn't you agree that our excellent mayor is doing a fantastic job?" (This question leads the respondent towards a positive answer.)
-
Unbiased: "What is your opinion of the mayor's performance?" (This question is neutral and allows for a range of responses.)
Another common pitfall is the use of complex or jargon-laden language. Questions should be clear, concise, and easily understood by all respondents, regardless of their background or level of education.
Furthermore, be mindful of social desirability bias. People tend to answer questions in a way that they believe will be viewed favorably by others. This can be particularly problematic when dealing with sensitive topics. Anonymity and confidentiality can help to mitigate this bias.
Participant Selection: Ensuring Representativeness
Even the most carefully worded questions can be undermined by a biased sample. If the participants in your survey are not representative of the target population, the results will be skewed.
-
Selection bias occurs when certain individuals or groups are systematically excluded from the sample. For example, if you conduct an online survey, you will inevitably exclude individuals who do not have access to the internet.
-
Self-selection bias arises when individuals voluntarily choose to participate in a survey. Those who choose to participate may have different characteristics or opinions than those who do not. For instance, individuals with strong opinions on a particular topic are more likely to participate in a survey about that topic.
To minimize these biases, it's essential to use a sampling method that provides all members of the target population with an equal opportunity to be selected. Random sampling is often the gold standard, but other techniques may be more appropriate depending on the specific research context.
The Power of Pilot Studies
Before launching a full-scale survey, it's always advisable to conduct a pilot study. A pilot study is a small-scale trial run that allows you to identify and address any potential problems with your survey design.
Pilot studies can help you:
-
Identify ambiguous or confusing questions.
-
Assess the clarity of response options.
-
Estimate the time required to complete the survey.
-
Detect any potential biases in the sampling method.
By conducting a pilot study, you can refine your survey design and increase the likelihood of obtaining accurate and reliable results. It's an investment that pays dividends in the form of higher-quality data and more informed decision-making.
Data Collection Crossroads: Navigating Methods and Minimizing Bias
Designing for Accuracy: Robust Survey Methodologies The margin of error is often presented as the ultimate arbiter of survey accuracy, a seemingly straightforward metric that quantifies the uncertainty inherent in sampling a larger population. However, placing undue faith in this statistic, particularly in the presence of underlying biases, is akin to navigating treacherous waters with a faulty compass. The chosen data collection method profoundly influences the presence and magnitude of such biases. Therefore, a critical evaluation of methodologies is paramount for reliable research.
A Comparative Look at Data Collection Methods
Selecting the appropriate data collection method is a pivotal decision in any survey research endeavor. Each approach – from online surveys to in-person interviews – presents unique advantages and disadvantages in terms of cost, reach, and, most importantly, susceptibility to bias. A rigorous comparative assessment is essential.
Online Surveys: Convenience at What Cost?
Online surveys offer unparalleled convenience and cost-effectiveness, enabling researchers to reach a geographically diverse audience with relative ease. However, this convenience comes with inherent risks.
The digital divide, for example, can introduce significant sampling bias, as individuals without internet access or digital literacy are systematically excluded. Furthermore, response rates for online surveys are often lower than those of other methods. This can lead to non-response bias if those who choose to participate differ systematically from those who do not.
Phone Surveys: A Diminishing Reach
Phone surveys, once a staple of survey research, face increasing challenges in the modern era. Declining response rates, driven by caller ID and call screening, present a substantial threat to representativeness.
Moreover, phone surveys are increasingly limited to reaching older demographics, potentially skewing results. While offering a higher degree of control over sample selection than online surveys, phone surveys are becoming less viable for general population studies.
In-Person Interviews: Depth and Detail, but at a Premium
In-person interviews offer the richest data and the opportunity for nuanced understanding, allowing researchers to probe for clarification and observe non-verbal cues. However, this method is the most expensive and time-consuming.
The potential for interviewer bias is also a concern, as the interviewer's demeanor and questioning style can inadvertently influence responses. Careful training and standardization are essential to mitigate this risk.
The Trade-Off Between Convenience and Representativeness
Researchers must carefully weigh the trade-offs between convenience and representativeness when selecting a data collection method. While online surveys offer the allure of speed and affordability, the potential for bias necessitates caution.
In contrast, in-person interviews, while resource-intensive, offer the greatest potential for minimizing bias and maximizing data quality.
The optimal choice depends on the specific research question, the target population, and the available resources. A well-reasoned justification for the chosen method is crucial for maintaining the credibility of the research.
The Role of Incentives: A Double-Edged Sword
Incentives, such as gift cards or monetary rewards, are frequently employed to boost survey response rates. While incentives can be effective in increasing participation, their impact on data quality is a subject of ongoing debate.
On one hand, incentives may attract a broader range of respondents, potentially reducing non-response bias. On the other hand, they may incentivize participation from individuals who are primarily motivated by the reward, rather than genuine interest in the topic.
This can lead to biased responses or even fraudulent data. Researchers must carefully consider the type and magnitude of incentives, as well as the potential impact on the validity of their findings. A pilot study to assess the effects of incentives is highly recommended.
Descriptive Statistics: Summarizing with Honesty and Clarity
Data collection, while critical, is only the initial step in the survey research process. The raw data gathered must then be distilled into a form that is both understandable and accurately reflects the characteristics of the sample. This is where descriptive statistics come into play, providing the tools to summarize and present data in a meaningful way. However, the selection and interpretation of these statistics must be approached with careful consideration of the data's distribution and potential biases.
Choosing the Right Summary Measure
Descriptive statistics provide a succinct snapshot of the key features of a dataset. The appropriate choice of statistic depends heavily on the nature of the data itself.
Measures of Central Tendency
The mean, or average, is perhaps the most commonly used measure of central tendency. It is calculated by summing all values and dividing by the number of values.
However, the mean is sensitive to outliers and may not be the best representation of the data if the distribution is skewed.
In such cases, the median, which is the middle value when the data is ordered, may provide a more robust measure of central tendency.
The mode, representing the most frequently occurring value, is particularly useful for categorical data.
Measures of Dispersion
While measures of central tendency tell us about the typical value, measures of dispersion describe the spread or variability of the data.
The standard deviation quantifies the average distance of each data point from the mean. A high standard deviation indicates greater variability.
Other measures of dispersion include variance, range, and interquartile range, each providing different insights into the distribution of the data.
The Shape Matters: Considering Data Distribution
The distribution of the data is a crucial factor in selecting appropriate descriptive statistics. Symmetrical distributions, such as the normal distribution, are well-described by the mean and standard deviation.
However, skewed distributions, where the data is concentrated on one side, require careful consideration.
For example, when analyzing income data, which is often right-skewed (i.e., a few high earners pull the mean upwards), the median is often a more representative measure of central tendency than the mean.
Ignoring the distribution can lead to misleading conclusions and a distorted understanding of the data.
Data Visualization: Telling the Story Clearly
Descriptive statistics are powerful tools, but their impact is amplified when combined with effective data visualization.
Histograms, box plots, and scatter plots can provide a visual representation of the data's distribution, highlighting patterns, outliers, and relationships that might be missed by numerical summaries alone.
Clear labeling and informative captions are essential to ensure that the visualizations are easily understood and accurately interpreted.
Misleading visualizations can distort the data and undermine the integrity of the research. It is thus important to use appropriate scaling, avoid unnecessary embellishments, and choose chart types that accurately represent the data.
In conclusion, descriptive statistics are essential for summarizing and presenting survey data. However, their effectiveness depends on choosing the appropriate measures, considering the distribution of the data, and using clear and informative visualizations. By approaching descriptive statistics with honesty and clarity, researchers can ensure that their findings are accurately communicated and effectively inform decision-making.
Media, Business, and Platforms: Unpacking Survey Influence and Bias
Data collection, while critical, is only the initial step in the survey research process. The raw data gathered must then be distilled into a form that is both understandable and accurately reflects the characteristics of the sample. This is where descriptive statistics come into play, providing a framework for summarizing and interpreting survey findings. However, the potential for bias extends far beyond the realm of statistical analysis. It permeates the very channels through which surveys are conducted, interpreted, and disseminated: media outlets, businesses with online platforms, and the online survey and social media platforms themselves.
The influence of these entities on survey data and the biases they introduce warrant careful scrutiny, underscoring the critical need for rigorous evaluation of data originating from these sources. Understanding these influences is crucial for interpreting survey results with a discerning eye.
The Media's Lens: Framing and Selective Reporting
Media outlets wield considerable power in shaping public opinion through their reporting of survey results. This influence, however, is not always exercised with impartiality. The way a survey is framed – the specific language used to describe the findings – can significantly impact how the audience perceives the data. For instance, highlighting a percentage increase in one area while downplaying a decrease in another can create a skewed impression of overall trends.
Furthermore, selective reporting – choosing to emphasize certain findings while omitting others – can further distort the picture. Media organizations may selectively highlight data that supports a particular narrative or agenda, leading to a biased representation of the survey's overall results. This selective presentation of data can inadvertently mislead the public.
Consider a poll on public support for a new environmental policy. A media outlet that favors the policy might emphasize the percentage of respondents who strongly support it. While simultaneously downplaying the percentage who oppose it or are undecided. This deliberate emphasis can create the impression of overwhelming public support. Even if the actual distribution of opinions is far more nuanced.
Business Platforms: Feedback Loops and Voluntary Response
Businesses increasingly rely on online platforms to gather feedback and conduct marketing surveys. These surveys, often integrated into user interfaces or disseminated through email campaigns, provide valuable insights into consumer preferences and behaviors. However, data collected through these channels is often subject to inherent biases.
Voluntary response bias is a primary concern. Individuals who choose to participate in these surveys are often those with particularly strong opinions, either positive or negative. This self-selection process can skew the results, making them unrepresentative of the broader customer base. For example, customers who have had a particularly positive or negative experience with a product are more likely to respond to a satisfaction survey.
Moreover, the design of the survey itself can introduce bias. Leading questions, poorly worded response options, or incentives offered for participation can all influence how respondents answer. A survey asking, "How satisfied are you with our excellent customer service?" is likely to elicit more positive responses than one simply asking, "How satisfied are you with our customer service?" The careful wording of survey questions is therefore crucial in order to avoid skewing the results.
Online Survey and Social Media Platforms: Echo Chambers and Data Quality
Online survey platforms have become indispensable tools for researchers across various disciplines. Social media platforms also offer avenues for gathering data through polls, quizzes, and user-generated content. However, these platforms are not without their challenges. Ensuring data quality and representativeness requires careful consideration.
One of the most significant challenges is the potential for echo chambers. Users on social media platforms often connect with like-minded individuals. Creating filter bubbles where they are primarily exposed to information that confirms their existing beliefs. When surveys are conducted within these echo chambers, the results may not accurately reflect the diversity of opinions in the broader population. This can create a skewed perception of public opinion.
Furthermore, data quality can be a concern. The anonymity afforded by online platforms can lead to respondents providing inaccurate or even fraudulent information. It is crucial to implement measures to verify respondent identities and ensure the integrity of the data collected. Researchers need to be vigilant about identifying and removing spurious responses.
In conclusion, the influence of media outlets, businesses with online platforms, and online survey and social media platforms on survey data cannot be ignored. These channels introduce potential biases that can significantly impact the validity and reliability of survey results. Critical evaluation, careful consideration of the data's source and context, and a healthy dose of skepticism are essential when interpreting survey findings from these sources. Only through such vigilance can we hope to glean meaningful insights from the ever-increasing flow of survey data in our modern world.
Ethical Imperatives: Transparency and Disclosure in Survey Research
The integrity of survey research hinges not only on rigorous methodology but also on ethical data interpretation and dissemination. Avoiding misleading or deceptive reporting is paramount, necessitating transparency and candid disclosure of any limitations inherent in the survey methodology. Responsible research demands a commitment to illuminating potential biases and their consequential impacts on the findings.
Upholding Ethical Obligations
At the heart of ethical survey research lies the obligation to present findings honestly and accurately. This extends beyond simply reporting the results to encompass a critical assessment of the data's strengths and weaknesses.
Misleading reporting, whether intentional or unintentional, can have severe consequences, distorting public understanding and influencing policy decisions based on flawed information.
Researchers must actively guard against selective reporting, cherry-picking data to support preconceived notions, or employing statistical manipulations that misrepresent the true nature of the findings.
Transparency in Methodology: A Cornerstone of Trust
Transparency in survey methodology is essential for fostering trust in the research process. This involves providing a detailed account of the survey's design, implementation, and analysis, enabling others to evaluate the validity and reliability of the findings.
This includes explicitly stating the sample size, sampling method, data collection procedures, and any potential sources of bias that may have influenced the results.
By providing this level of transparency, researchers empower others to critically assess the research and draw their own informed conclusions.
Addressing Limitations Head-On
No survey is perfect, and every study will have limitations. Acknowledging these limitations is not a sign of weakness but rather a mark of intellectual honesty.
Researchers should proactively identify and discuss any potential sources of bias, such as sampling bias, response bias, or measurement error. They should also assess the potential impact of these limitations on the interpretation of the findings.
By transparently addressing limitations, researchers provide a more complete and nuanced picture of the research, allowing readers to make informed judgments about the validity and generalizability of the results.
Communicating Uncertainty to a General Audience
Communicating the inherent uncertainty of survey findings to a non-technical audience presents a unique challenge. Statistical concepts such as confidence intervals and margins of error can be difficult for the general public to grasp.
Researchers must strive to present this information in a clear, concise, and accessible manner, avoiding technical jargon and focusing on the practical implications of the findings.
Using visual aids, such as graphs and charts, can be helpful in illustrating the range of possible values and the degree of uncertainty associated with the estimates.
It is also important to emphasize that survey findings are not definitive truths but rather estimates based on a sample of the population.
By effectively communicating uncertainty, researchers can help ensure that the public understands the limitations of survey research and avoids drawing unwarranted conclusions. This fosters a more informed and discerning public discourse, grounded in realistic expectations of the data.
Purpose-Driven Sampling: Aligning Methods with Research Goals
[Ethical Imperatives: Transparency and Disclosure in Survey Research The integrity of survey research hinges not only on rigorous methodology but also on ethical data interpretation and dissemination. Avoiding misleading or deceptive reporting is paramount, necessitating transparency and candid disclosure of any limitations inherent in the survey methodology. Building on these ethical considerations, we now turn to the critical process of purpose-driven sampling and aligning methods with research goals to ensure that the effort invested in the research yields genuinely meaningful results.]
The effectiveness of any sampling endeavor hinges upon a clear articulation of its intended goal. This foundational step dictates the subsequent decision-making process, shaping the selection of appropriate methodologies and influencing the ultimate interpretability of the findings. A disconnect between the research objective and the sampling strategy can lead to skewed results, undermining the validity and relevance of the entire study.
The Primacy of Research Aims
Consider a scenario where the objective is to gauge overall customer satisfaction with a new product. A random sampling of all customers would be a suitable approach.
Conversely, if the goal is to understand why a segment of customers is dissatisfied, a targeted sampling strategy focusing on those who have registered complaints or returned the product would be more appropriate.
Each research objective necessitates a tailored sampling approach.
Defining the Research Question: A Compass for Sampling
A well-defined research question serves as a compass, guiding the sampling process and ensuring that the collected data directly addresses the core inquiry. The research question dictates the target population, the relevant variables, and the level of precision required in the sample estimates.
Ambiguity in the research question translates to uncertainty in the sampling strategy.
Impact on Generalizability
The choice of sampling strategy has a profound impact on the generalizability of the findings. Probability sampling methods, such as simple random sampling or stratified sampling, allow for statistical inferences to be drawn about the entire population from which the sample was selected.
Non-probability sampling methods, such as convenience sampling or snowball sampling, may be useful for exploratory research or for reaching specific subpopulations. However, caution must be exercised in generalizing findings beyond the sample itself.
Examples of Strategies for Research Goals
To illustrate further, let's explore several examples:
- Exploratory Research: If the goal is to explore a new research area or generate hypotheses, convenience sampling might be acceptable. This method quickly gathers initial insights but offers limited generalizability.
- In-Depth Qualitative Research: In cases where detailed insights are needed from a specific subgroup, purposive sampling can be used. Researchers deliberately select participants based on predefined characteristics.
- Estimating Population Parameters: When the objective is to estimate population parameters like means or proportions, probability sampling is crucial. It ensures that each member of the population has a known, non-zero chance of being selected, enabling unbiased estimates.
- Longitudinal Studies: For research tracking changes over time, panel sampling is used, following the same participants at multiple points in time. This provides valuable insights into trends and individual trajectories.
The Iterative Nature of Sampling
Sampling is not merely a preliminary step. It's an iterative process that may require refinement as the research progresses. Pilot studies can help identify potential biases or limitations in the initial sampling strategy, allowing for adjustments to be made before the main data collection effort.
Regularly reassessing the alignment between the sampling method and the research objectives is essential throughout the study.
Video: Voluntary Response Sampling: Pros, Cons & Examples
FAQs about Voluntary Response Sampling
What is the biggest problem with voluntary response sampling?
The primary issue with voluntary response sampling is its inherent bias. People who choose to participate in a survey or study typically have strong opinions, often negative, about the subject matter, leading to results that are not representative of the broader population. This skews the data, making it unreliable for drawing general conclusions.
What are some common scenarios where voluntary response sampling is used?
Voluntary response sampling is frequently used in online polls, call-in surveys for radio or television programs, and customer feedback forms. These methods rely on individuals self-selecting to participate, meaning the results may reflect the views of a vocal minority rather than the average consumer or citizen.
What is an example of a positive use case for voluntary response sampling?
While generally problematic, voluntary response sampling can be useful for quickly gathering anecdotal feedback or identifying extreme opinions. For example, a company might use it to gauge initial reactions to a product change, understanding that the feedback will not be statistically representative, but can highlight potential major concerns.
How does voluntary response sampling differ from random sampling?
Unlike voluntary response sampling, random sampling methods ensure every member of the population has an equal chance of being selected. This reduces bias and allows for more accurate inferences about the entire population. Voluntary response sampling offers no such guarantee, as participation is self-selected.
So, there you have it – the good, the bad, and the sometimes-ugly truth about voluntary response sampling. It's a quick and easy method, but remember that the results should always be taken with a grain of salt. Understanding its limitations is key before using voluntary response sampling in your next survey or study!