P Wave Inversion: Master the Technique for Better Results
The field of seismic interpretation heavily relies on accurate subsurface models. One crucial technique for refining these models involves p wave inversion, a method particularly important in oil and gas exploration. Understanding the nuances of p wave inversion workflows, especially within software like Petrel, allows geophysicists to derive detailed acoustic impedance models. These models, in turn, aid in reservoir characterization, a key area of expertise for individuals like Dr. John Doe, a renowned expert. By mastering the technique for p wave inversion, practitioners can achieve significantly better results in subsurface imaging, improving the precision of exploration.
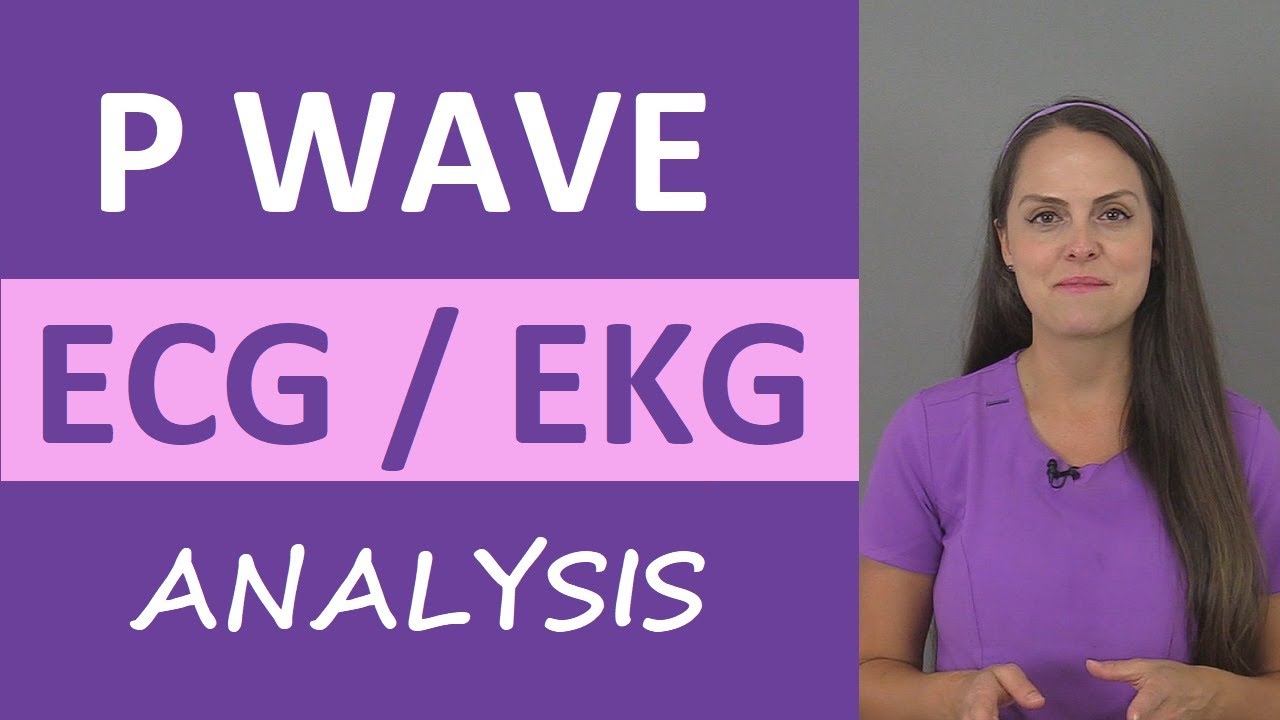
Image taken from the YouTube channel RegisteredNurseRN , from the video titled P Wave ECG EKG Heart Rhythm Analysis Interpretation Nursing NCLEX Cardiac .
Unveiling the Power of P-Wave Inversion: A Comprehensive Guide
P-Wave Inversion stands as a cornerstone technique in modern geophysics, offering invaluable insights into the Earth's subsurface. It transcends the limitations of raw seismic data, transforming it into a detailed representation of subsurface rock properties. This transformation unlocks a deeper understanding of geological formations, fluid content, and ultimately, the potential of hydrocarbon reservoirs.
This article aims to provide a thorough understanding of P-Wave Inversion, exploring its principles, methodologies, and applications. Whether you are a seasoned geoscientist or a newcomer to the field, this guide will equip you with the knowledge to harness the power of P-Wave Inversion effectively. By mastering this technique, you can significantly enhance reservoir analysis, optimize exploration strategies, and minimize risks in subsurface endeavors.
Defining P-Wave Inversion
At its core, P-Wave Inversion is a process that estimates subsurface acoustic impedance from seismic reflection data. Acoustic impedance, a fundamental rock property, is the product of seismic velocity and density. Variations in acoustic impedance cause seismic reflections, which are the raw data for inversion.
The process involves using sophisticated algorithms to convert seismic amplitudes into quantitative estimates of acoustic impedance. This is not a direct measurement, but rather an inversion – a mathematical process of working backward from the observed data to infer the underlying properties.
The resulting acoustic impedance model provides a detailed picture of subsurface layering, lithology variations, and fluid content. This model is critical for reservoir characterization, as it provides insights into key properties like porosity, permeability, and fluid saturation.
The Significance of P-Wave Inversion
P-Wave Inversion plays a pivotal role in both reservoir characterization and exploration, significantly impacting decision-making in the oil and gas industry.
In reservoir characterization, P-Wave Inversion refines reservoir models, leading to more accurate estimations of crucial parameters. It assists in identifying potential sweet spots within the reservoir, optimizing well placement, and improving production forecasts.
In exploration, P-Wave Inversion is crucial for de-risking exploration drilling. By providing a more accurate subsurface image, it helps identify potential hydrocarbon traps and reduces the uncertainty associated with exploration ventures. It allows geoscientists to make informed decisions about where to drill, increasing the chances of success and minimizing wasted resources.
What You Will Gain From This Guide
This article will delve into the intricacies of P-Wave Inversion, taking you on a journey from the fundamental concepts to practical applications. Readers will develop a strong understanding of:
-
The underlying principles of P-Wave Inversion and its relationship to rock properties.
-
The various steps involved in the P-Wave Inversion workflow, from data preparation to result interpretation.
-
The different types of P-Wave Inversion methods and their respective advantages and disadvantages.
-
The importance of integrating P-Wave Inversion with other geophysical techniques, such as Rock Physics and AVO analysis.
-
Real-world case studies demonstrating the successful application of P-Wave Inversion in reservoir characterization and exploration.
By the end of this guide, you will possess the knowledge and skills to effectively utilize P-Wave Inversion in your own subsurface characterization endeavors.
The Foundation: Understanding Acoustic Impedance, Velocity Modeling, and Seismic Data
As we embark on understanding P-Wave Inversion, it's critical to establish a firm grasp on the foundational concepts that underpin the entire process. These building blocks include acoustic impedance, velocity modeling, and seismic data. Each plays a vital role in transforming raw seismic reflections into meaningful subsurface information. Without a solid understanding of these fundamentals, the complexities of P-Wave Inversion can become overwhelming, hindering the ability to interpret results accurately.
Acoustic Impedance: The Cornerstone
At the heart of P-Wave Inversion lies acoustic impedance (AI), a fundamental rock property that governs how seismic waves interact with subsurface formations. It's defined as the product of a rock's density and P-wave velocity:
AI = Density x P-wave Velocity.
This seemingly simple relationship is the key to unlocking subsurface geological information.
The Significance of Acoustic Impedance Contrasts
Variations in acoustic impedance create impedance contrasts between different rock layers. When a seismic wave encounters an impedance contrast, a portion of its energy is reflected back to the surface, forming the seismic reflections that we observe. The magnitude of the reflection is directly related to the size of the impedance contrast.
Therefore, mapping these reflections allows us to infer changes in rock properties. High impedance contrasts may indicate boundaries between different lithologies (e.g., sandstone and shale), or changes in fluid content (e.g., a gas-water contact). This relationship is the foundation upon which P-Wave Inversion is built.
Velocity Modeling: Building the Framework
Accurate velocity modeling is paramount for effective P-Wave Inversion. The velocity model serves as the backbone for depth conversion and seismic imaging, significantly influencing the accuracy of the final inversion results. An incorrect velocity model can lead to mispositioned reflectors, distorted subsurface structures, and ultimately, inaccurate impedance estimates.
Methods for Constructing Velocity Models
Several techniques are used to build velocity models, each with its strengths and limitations:
-
Well Logs: Well logs provide direct measurements of velocity at specific borehole locations. These data offer high vertical resolution but are limited to the vicinity of the well.
-
Seismic Tomography: Seismic tomography uses travel times of seismic waves to create a 3D velocity model. This method provides broader coverage but can be less accurate than well logs.
-
Traveltime Inversion: Traveltime inversion is a technique that uses the arrival times of reflected or refracted seismic waves to estimate subsurface velocities.
The Perils of Inaccurate Velocity Models
Inaccurate velocity models can have a detrimental impact on P-Wave Inversion. Distorted subsurface images, mispositioned reflectors, and erroneous impedance estimates can all be consequences of velocity model errors. Inaccurate velocity models introduce errors in time-to-depth conversion. This directly affects the accuracy of the resulting impedance model. It is crucial to invest effort in constructing a reliable velocity model.
Seismic Data: The Raw Material
Seismic data forms the raw material for P-Wave Inversion. It comprises recordings of seismic waves that have traveled through the Earth and reflected back to the surface. The quality and characteristics of seismic data profoundly influence the success of any inversion project.
Types of Seismic Data
Different types of seismic data can be used for P-Wave Inversion, including:
- 2D Seismic: 2D seismic data provides a cross-sectional view of the subsurface along a single line.
- 3D Seismic: 3D seismic data provides a volumetric representation of the subsurface, offering a more comprehensive view of geological structures.
- 4D Seismic: 4D seismic data involves repeated 3D seismic surveys over time to monitor changes in the reservoir, such as fluid movement during production.
The Importance of Seismic Data Processing
Seismic data requires careful processing to enhance its quality and prepare it for inversion. Several key processing steps are crucial:
- Noise Reduction: Noise reduction techniques aim to remove unwanted signals from the seismic data, improving the signal-to-noise ratio.
- Multiple Removal: Multiple reflections, which are seismic waves that have been reflected multiple times within the subsurface, can obscure primary reflections. Multiple removal techniques are used to attenuate these unwanted signals.
- Amplitude Preservation: Amplitude preservation is essential for P-Wave Inversion, as the amplitudes of seismic reflections are directly related to acoustic impedance contrasts. Processing workflows should be designed to preserve these amplitudes as accurately as possible.
Proper seismic data processing is an essential prerequisite for successful P-Wave Inversion. Without high-quality, properly processed data, the inversion results will likely be unreliable.
The P-Wave Inversion Process: A Step-by-Step Guide
Having established the fundamental building blocks of acoustic impedance, velocity modeling, and seismic data, we can now delve into the heart of the matter: the P-Wave Inversion process itself. This section will dissect the workflow, comparing pre-stack and post-stack approaches, and emphasize the crucial role of well log integration.
Unveiling the P-Wave Inversion Workflow
P-Wave Inversion is not a "black box" solution, but rather a carefully orchestrated sequence of steps. Understanding this workflow is essential for achieving reliable and meaningful results.
The process generally involves:
-
Data Loading and Preparation: The first step involves gathering and preparing the necessary data, which typically includes seismic data and well logs. Seismic data requires careful quality control, ensuring proper geometry and amplitude fidelity. Well logs need to be checked for accuracy and completeness, with necessary environmental corrections applied.
-
Initial Model Building: An initial model is required as a starting point for the inversion process. This model usually consists of a velocity model and an initial impedance model. The velocity model can be derived from well logs, stacking velocities, or tomographic inversion. The initial impedance model can be built using the velocity model and a smoothed version of the seismic data or a low-frequency impedance model derived from well logs.
-
Inversion Execution and Parameter Tuning: This is where the inversion algorithm works its magic. The algorithm iteratively modifies the impedance model to minimize the difference between the synthetic seismic data generated from the model and the real seismic data. Parameter tuning is crucial here, as the choice of algorithm, regularization parameters, and constraints can significantly impact the results.
-
Post-Inversion Analysis and Validation: Once the inversion is complete, the resulting impedance model needs to be thoroughly analyzed and validated. This involves comparing the inverted impedance with well logs, geological information, and other independent data sources. Quality control metrics, such as the data misfit and model smoothness, should also be examined.
Pre-stack vs. Post-stack Inversion: Choosing the Right Approach
One of the key decisions in P-Wave Inversion is whether to use a pre-stack or post-stack approach. Each has its own set of advantages and disadvantages.
Pre-stack Inversion: A Deep Dive
Pre-stack inversion operates directly on seismic gathers, which are collections of traces recorded at different offsets (distances between the source and receivers). This allows for the incorporation of Amplitude Variation with Offset (AVO) information, making it more sensitive to lithology and fluid content.
Advantages:
- Enhanced Lithology Discrimination: AVO analysis is key to differentiating lithology.
- Fluid Sensitivity: Detects changes in fluids with greater sensitivity.
- More Accurate Results in Complex Geology: Can handle complex structures.
Disadvantages:
- Higher Computational Cost: Requires greater computing power.
- More Demanding Data Requirements: Demands high-quality seismic data.
- Greater Sensitivity to Noise: Noise greatly affects data quality.
Pre-stack inversion is best suited for projects where detailed lithological information and fluid identification are critical, and where high-quality seismic data is available. It is particularly valuable in AVO analysis.
Post-stack Inversion: A Simplified View
Post-stack inversion, on the other hand, operates on stacked seismic data, which is a single trace representing the average of all traces at a given location. This simplification reduces computational cost but also eliminates AVO information.
Advantages:
- Lower Computational Cost: Does not demand too much computer power.
- Less Demanding Data Requirements: Can work well with lower data quality.
- Less Sensitivity to Noise: Post-stacking reduces noise impacts.
Disadvantages:
- Reduced Lithology Discrimination: Limited capacity to differentiate rock types.
- Limited Fluid Sensitivity: Limited capacity to determine fluid changes.
- Less Accurate Results in Complex Geology: Struggles with complex structures.
Post-stack inversion is appropriate for projects where computational resources are limited, data quality is poor, or the primary goal is to delineate gross structural features.
In essence, the choice between pre-stack and post-stack inversion hinges on a trade-off between accuracy, detail, and computational cost.
Integrating Well Logs for Calibration and Interpretation
Well logs provide direct measurements of subsurface rock properties at specific locations. Integrating them with seismic data is essential for calibrating and validating P-Wave Inversion results.
The Importance of Well Log Correlation
Well log correlation is the process of comparing and aligning well logs from different wells to identify geological formations and correlate their properties. This provides a framework for understanding the subsurface geology and for tying seismic data to well control.
Tying Seismic Data to Well Logs
One of the most common techniques for tying seismic data to well logs is the generation of synthetic seismograms. A synthetic seismogram is a modeled seismic trace created by convolving a reflectivity series (derived from well logs) with a wavelet (representing the seismic source).
By comparing the synthetic seismogram with the real seismic data at the well location, we can:
- Verify the accuracy of the velocity model
- Identify the seismic reflections corresponding to specific geological formations
- Calibrate the amplitude scale of the seismic data
- Validate the P-Wave Inversion results
Without proper well log integration, the interpretation of P-Wave Inversion results becomes highly speculative. Well logs provide the ground truth necessary to transform inverted impedance models into meaningful geological insights.
Having navigated the step-by-step process of P-Wave Inversion, it's time to consider how we can refine our results and extract even more geological insight. The accuracy and reliability of P-Wave Inversion can be significantly improved by integrating complementary techniques. Let's explore how incorporating Rock Physics models and Amplitude Variation with Offset (AVO) analysis can elevate your P-Wave Inversion from a good result to a truly exceptional one.
Enhancing Inversion with Rock Physics and AVO Analysis
P-Wave Inversion provides a detailed picture of acoustic impedance variations in the subsurface. However, to translate impedance values into meaningful geological parameters like porosity, lithology, and fluid saturation, we need additional constraints and insights. This is where the power of Rock Physics and AVO analysis comes into play, enriching the inversion process and unlocking a more complete understanding of the reservoir.
Rock Physics: Constraining the Solution Space
Rock Physics provides the critical link between seismic data and reservoir properties. It establishes quantitative relationships between acoustic impedance and reservoir characteristics such as porosity, lithology, and fluid saturation. Without Rock Physics, P-Wave Inversion alone can yield multiple possible solutions, making it difficult to uniquely determine reservoir properties.
By incorporating Rock Physics models into the inversion process, we can significantly narrow down the range of plausible solutions. This is because Rock Physics models provide mathematical constraints that relate impedance to specific combinations of reservoir properties.
Building and Calibrating Rock Physics Models
The first step is to build a Rock Physics model that is appropriate for the specific geological setting. This involves selecting appropriate theoretical models or empirical relationships. These models take into account factors such as mineralogy, pore shape, and fluid properties.
Once a model is built, it needs to be calibrated using well log data. This ensures that the model accurately reflects the local geological conditions.
The calibration process involves comparing predicted impedance values from the Rock Physics model to the actual impedance values measured in the well logs.
Any discrepancies between the model predictions and the well log data can be used to refine the model parameters and improve its accuracy.
The Impact of Rock Physics on Inversion
Incorporating Rock Physics models into P-Wave Inversion can significantly improve the accuracy and reliability of the results. By using Rock Physics as a constraint, the inversion algorithm can focus on solutions that are consistent with both the seismic data and the known relationships between impedance and reservoir properties. This leads to more reliable estimations of porosity, lithology, and fluid saturation, which are crucial for reservoir characterization and management.
AVO Analysis: Adding Lithological Sensitivity
Amplitude Variation with Offset (AVO) analysis exploits the changes in seismic reflection amplitude with increasing source-receiver offset. These amplitude variations are directly related to changes in P-wave velocity (Vp), S-wave velocity (Vs), and density (ρ) across a reflecting interface. This provides additional information about the lithology and fluid content of the rocks.
Common AVO Attributes and Their Interpretation
Several AVO attributes can be derived from the seismic data, each sensitive to different rock properties. Some of the most common attributes include:
- Intercept (A): Represents the reflection amplitude at zero offset, primarily related to the change in acoustic impedance.
- Gradient (B): Measures the change in reflection amplitude with increasing offset. It is sensitive to the Vp/Vs ratio.
- AVO Intercept Gradient (AB): A commonly used attribute that combines the intercept and gradient. This helps to distinguish between different lithologies and fluid types.
By analyzing these attributes, geoscientists can identify potential hydrocarbon reservoirs.
Integrating AVO Analysis with P-Wave Inversion
Integrating AVO analysis with P-Wave Inversion can significantly improve lithology discrimination. AVO attributes can be used as additional constraints in the inversion process, guiding the algorithm towards solutions that are consistent with both the seismic data and the AVO response.
This approach is particularly useful in complex geological settings where traditional P-Wave Inversion alone may not provide sufficient resolution. By incorporating AVO information, we can better differentiate between different lithologies. We can also improve our ability to identify potential hydrocarbon reservoirs.
Having seen how Rock Physics and AVO analysis can augment P-Wave Inversion, allowing for a more nuanced understanding of subsurface properties, it's time to examine how these techniques translate into tangible results in the field. Let's ground our discussion with real-world examples, showcasing the practical applications and impact of P-Wave Inversion on reservoir characterization and exploration.
Real-World Applications: Case Studies in Reservoir Characterization and Exploration
P-Wave Inversion is not merely a theoretical exercise; it is a powerful tool that has been successfully deployed in numerous reservoir characterization and exploration projects around the globe. These applications demonstrate its ability to refine reservoir models, de-risk exploration drilling, and ultimately, improve decision-making in the oil and gas industry. Let's delve into specific examples that illustrate the transformative potential of this technique.
Refining Reservoir Models with P-Wave Inversion
One of the most significant benefits of P-Wave Inversion lies in its ability to refine reservoir models. Traditional methods often rely on sparse well data and broad geological assumptions, leading to uncertainties in key reservoir parameters. P-Wave Inversion, however, provides a high-resolution 3D image of acoustic impedance, which can be directly related to reservoir properties through Rock Physics models.
Improved Estimation of Key Reservoir Parameters:
P-Wave Inversion enhances the estimation of:
- Porosity: By establishing a relationship between acoustic impedance and porosity, inversion allows for a more accurate mapping of porosity distribution throughout the reservoir.
- Net-to-Gross: Accurate impedance contrasts can delineate reservoir boundaries and identify non-reservoir intervals, leading to improved net-to-gross estimations.
- Fluid Saturation: Integrating Rock Physics models and AVO analysis with P-Wave Inversion allows for a better understanding of fluid distribution and saturation levels within the reservoir.
These refined estimations are crucial for accurate reservoir modeling, which in turn, supports better production forecasting and optimized field development strategies.
De-Risking Exploration Drilling with Enhanced Subsurface Information
Exploration drilling is inherently risky, with high upfront costs and a significant chance of failure. P-Wave Inversion can play a vital role in de-risking these operations by providing more accurate subsurface information and identifying potential hydrocarbon traps before drilling commences.
By providing a higher resolution picture of subsurface structures and rock properties than conventional seismic interpretation alone, P-Wave Inversion helps in:
- Identifying Subtle Traps: Inversion can reveal subtle structural and stratigraphic traps that might be missed by traditional seismic interpretation.
- Predicting Reservoir Presence: By mapping acoustic impedance variations and relating them to reservoir properties, P-Wave Inversion helps predict the presence and extent of reservoir facies.
- Assessing Fluid Content: Integrating AVO analysis with P-Wave Inversion allows for the identification of potential hydrocarbon-bearing zones, reducing the risk of drilling dry holes.
Case Study Examples
While the general benefits of P-Wave Inversion are clear, specific case studies provide a more concrete understanding of its impact. Here are a few examples:
- North Sea Turbidite Reservoir: P-Wave Inversion was used to map the distribution of sand-rich turbidite channels within a complex reservoir system. This allowed for a more accurate estimation of reservoir volume and improved placement of development wells.
- Gulf of Mexico Deepwater Exploration: AVO analysis, integrated with P-Wave Inversion, was instrumental in identifying a subtle hydrocarbon trap in a deepwater setting. This led to a successful exploration well and the discovery of a new oil field.
- Onshore Shale Gas Reservoir: P-Wave Inversion was used to characterize the heterogeneity of a shale gas reservoir, identifying areas of high organic content and improved fracture density. This helped optimize hydraulic fracturing operations and improve gas production rates.
These examples highlight the diverse range of applications for P-Wave Inversion, demonstrating its ability to address various challenges in both reservoir characterization and exploration.
By carefully considering the geological setting, selecting appropriate data, and tuning inversion parameters, geoscientists can leverage the power of P-Wave Inversion to unlock valuable insights and improve decision-making in the oil and gas industry.
Having seen how Rock Physics and AVO analysis can augment P-Wave Inversion, allowing for a more nuanced understanding of subsurface properties, it's time to examine how these techniques translate into tangible results in the field. Let's ground our discussion with real-world examples, showcasing the practical applications and impact of P-Wave Inversion on reservoir characterization and exploration.
Software Toolkit: Exploring Popular P-Wave Inversion Platforms
The implementation of P-Wave Inversion relies heavily on specialized software. These platforms provide the necessary tools and workflows to process seismic data, build models, execute inversion algorithms, and interpret results. Choosing the right software is crucial for efficient and accurate subsurface characterization.
Let's explore some of the leading software packages used in the industry, examining their key features and functionalities.
Leading Platforms for P-Wave Inversion
Several software packages dominate the market for P-Wave Inversion, each offering a unique set of capabilities and strengths. Here, we will focus on two prominent platforms: Petrel (Schlumberger) and HampsonRussell (CGG).
Petrel (Schlumberger)
Petrel is a comprehensive geoscience software platform widely used in the oil and gas industry. Its strength lies in its integrated environment, which allows users to seamlessly move between different workflows, including seismic interpretation, geological modeling, reservoir simulation, and, of course, P-Wave Inversion.
Key Features and Workflows:
-
Comprehensive Inversion Modules: Petrel offers a range of inversion algorithms, including pre-stack and post-stack methods.
-
Seamless Integration: Its tight integration with other Petrel modules allows for easy data sharing and workflow automation. Velocity models built in Petrel's velocity modeling module can be directly used as input for inversion.
-
Rock Physics Integration: Petrel provides tools for Rock Physics modeling, allowing users to establish relationships between acoustic impedance and reservoir properties.
-
Visualization and Interpretation: Petrel's powerful visualization capabilities enable users to interpret inversion results in 3D and integrate them with other subsurface data.
Integration with Other Petrel Modules:
The true power of Petrel lies in its ability to connect different workflows. For example, seismic interpretations made in Petrel's seismic interpretation module can be used to guide the inversion process. Similarly, reservoir models built in Petrel's reservoir modeling module can be updated with inversion results to improve their accuracy.
HampsonRussell (CGG)
HampsonRussell, now part of CGG, is another popular software suite for seismic interpretation and reservoir characterization. It is particularly known for its user-friendly interface and its focus on advanced seismic analysis techniques, including AVO analysis and P-Wave Inversion.
Key Features and Workflows:
-
Dedicated Inversion Modules: HampsonRussell offers specialized modules for different types of inversion, including pre-stack and post-stack inversion.
-
User-Friendly Interface: The software is known for its intuitive interface, making it easier for users to learn and use.
-
AVO Analysis Tools: HampsonRussell has strong capabilities for AVO analysis, which can be integrated with P-Wave Inversion to improve lithology discrimination.
-
Rock Physics Integration: Similar to Petrel, HampsonRussell allows users to integrate Rock Physics models into the inversion workflow.
Integration with Other HampsonRussell Modules:
HampsonRussell's modules are designed to work together seamlessly. For instance, AVO attributes calculated in the AVO module can be used as input for the inversion process. Likewise, inversion results can be used to update reservoir models built in HampsonRussell's reservoir characterization module.
Other Relevant Software
While Petrel and HampsonRussell are leading platforms, other software packages also offer P-Wave Inversion capabilities. These include:
-
SeisSpace (Landmark/Halliburton): Another comprehensive geoscience software platform.
-
Geoteric: Known for its advanced seismic attribute analysis and visualization capabilities.
-
Open Source Options: Some open-source software packages, such as Seismic Unix, can be used for basic P-Wave Inversion tasks, although they typically require more programming expertise.
Software Comparison: Ease of Use, Functionality, and Cost
Choosing the right software depends on specific project requirements, budget constraints, and user preferences. Here's a brief comparison of the key factors to consider:
-
Ease of Use: HampsonRussell is generally considered more user-friendly, while Petrel can have a steeper learning curve due to its complexity.
-
Functionality: Petrel offers a broader range of functionalities due to its integrated environment, while HampsonRussell focuses on advanced seismic analysis.
-
Cost: The cost of software licenses can vary significantly. Open-source options are free, while commercial software packages like Petrel and HampsonRussell can be expensive. Consider the long-term costs of training, maintenance, and upgrades.
Ultimately, the best software is the one that best meets the needs of the project and the skills of the users.
Having dedicated time and effort to P-Wave Inversion, securing reliable results hinges on diligent practices. Overlooking the essential steps of data quality control and model validation can undermine the entire process, leading to inaccurate subsurface interpretations and potentially flawed decision-making. Let's delve into the critical aspects of avoiding common pitfalls in P-Wave Inversion, ensuring that your efforts yield trustworthy and actionable insights.
Avoiding the Pitfalls: Best Practices for Reliable Results
Successful P-Wave Inversion demands not only expertise in the process itself but also a keen awareness of potential pitfalls that can compromise the accuracy and reliability of the results. This section focuses on two crucial aspects: rigorous data quality assessment and thorough model validation.
Data Quality Assessment: The Foundation of Success
Before embarking on P-Wave Inversion, a thorough assessment of data quality is paramount. Garbage in, garbage out is a fitting adage.
Compromised data quality can propagate errors throughout the inversion process, leading to inaccurate estimations of subsurface properties. This phase is not merely a formality; it's the bedrock upon which the entire inversion endeavor rests.
Common Data Quality Issues
Several factors can degrade the quality of seismic data. Recognizing and addressing these issues is critical.
- Noise: Random or coherent noise can obscure the true seismic signal, affecting the accuracy of amplitude and traveltime estimations.
- Multiples: Multiples are reflections that have bounced multiple times within the subsurface, creating false events that interfere with primary reflections.
- Poor Signal-to-Noise Ratio (SNR): A low SNR makes it difficult to distinguish meaningful seismic signals from background noise.
Techniques for Addressing Data Quality Issues
Fortunately, various techniques exist to mitigate data quality issues. These techniques must be applied judiciously.
- Noise Reduction: Techniques like filtering, stacking, and Radon transforms can be employed to suppress noise.
- Multiple Removal: Predictive deconvolution and surface-related multiple elimination (SRME) are common methods for attenuating multiples.
- Careful Data Processing: Paying close attention to data processing steps like amplitude preservation is important.
Model Validation: Ensuring Accuracy and Reliability
Even with high-quality data, the inversion results must be rigorously validated to ensure their accuracy and reliability. Validation is not an optional step, but an integral part of the inversion workflow.
This involves comparing the inversion results with independent data sources to assess their consistency and identify potential discrepancies.
Independent Data Sources for Validation
Several independent data sources can be used to validate inversion results. These sources provide a reality check.
- Well Logs: Well logs provide direct measurements of subsurface properties at specific locations.
- Core Data: Core samples provide valuable information about lithology, porosity, and fluid saturation.
- Production Data: Production data, such as oil and gas flow rates, can provide insights into reservoir connectivity and permeability.
- Analogous Field Data: Data from similar fields or geological settings can provide valuable constraints on the expected range of reservoir properties.
Techniques for Comparing Inversion Results to Well Logs
Comparing inversion results to well logs is a crucial step in the validation process.
- Synthetic Seismograms: Generate synthetic seismograms from well logs and compare them to the real seismic data to ensure proper time-depth alignment.
- Cross-plotting: Cross-plot inversion-derived properties (e.g., Acoustic Impedance) against well log measurements (e.g., porosity) to assess their correlation.
- Quantitative Comparison: Calculate statistical measures (e.g., correlation coefficient, root mean squared error) to quantify the agreement between inversion results and well log data.
Identifying and Mitigating Potential Errors
Discrepancies between inversion results and independent data sources may indicate errors in the inversion process.
It is crucial to investigate these discrepancies and identify their root causes. Potential sources of error include:
- Inaccurate Velocity Model: An inaccurate velocity model can lead to mis-positioning of seismic events and errors in the inversion results.
- Poor Initial Model: The initial impedance model can significantly influence the final inversion results.
- Inappropriate Inversion Parameters: Selecting inappropriate inversion parameters can lead to suboptimal results.
By carefully evaluating data quality and rigorously validating inversion results, you can minimize the risk of errors and ensure that your P-Wave Inversion efforts provide reliable and actionable insights for subsurface characterization and exploration.
Video: P Wave Inversion: Master the Technique for Better Results
Frequently Asked Questions About P-Wave Inversion
Here are some common questions about P-wave inversion to help you better understand this powerful technique.
What exactly is P-wave inversion?
P-wave inversion is a geophysical technique used to estimate subsurface rock properties, such as acoustic impedance, from seismic data. It helps convert seismic reflections into a more interpretable geological model. Understanding the nuances of P-wave inversion is crucial for accurate reservoir characterization.
Why is acoustic impedance so important in P-wave inversion?
Acoustic impedance, which is the product of velocity and density, is the primary property derived from P-wave inversion. It's crucial because it's directly related to lithology, porosity, and fluid content in subsurface formations. Accurate impedance models, obtained through P-wave inversion, are key to understanding reservoir characteristics.
What are the key steps involved in a typical P-wave inversion workflow?
The common workflow usually includes data conditioning, wavelet estimation, building an initial model (using well logs and geological information), and then the inversion process itself. After inversion, the resulting impedance model is then interpreted geologically. Each step is important to obtaining the most accurate P-wave inversion results.
What are some potential challenges or limitations of P-wave inversion?
Challenges may include poor data quality (noise, multiples), inaccurate wavelet estimation, and limitations in the initial model. The reliability of P-wave inversion results depends heavily on the quality of input data and the accuracy of the geological model used to guide the process. Overcoming these challenges is important for successful P-wave inversion outcomes.