Manipulated Variable: Definition & Examples
In experimental design, researchers often utilize the scientific method, a systematic approach to inquiry, where the manipulated variable is a central component. Experiments, frequently conducted in laboratory settings or in real-world conditions like those studied by organizations such as the National Science Foundation (NSF), depend on the researcher's ability to control and change independent variables. The fundamental characteristic of the manipulated variable is its intentional alteration by the experimenter to observe its effects on the dependent variable, a concept closely monitored using statistical analysis tools. Understanding the role and application of the manipulated variable is crucial for drawing valid conclusions and building evidence-based knowledge.
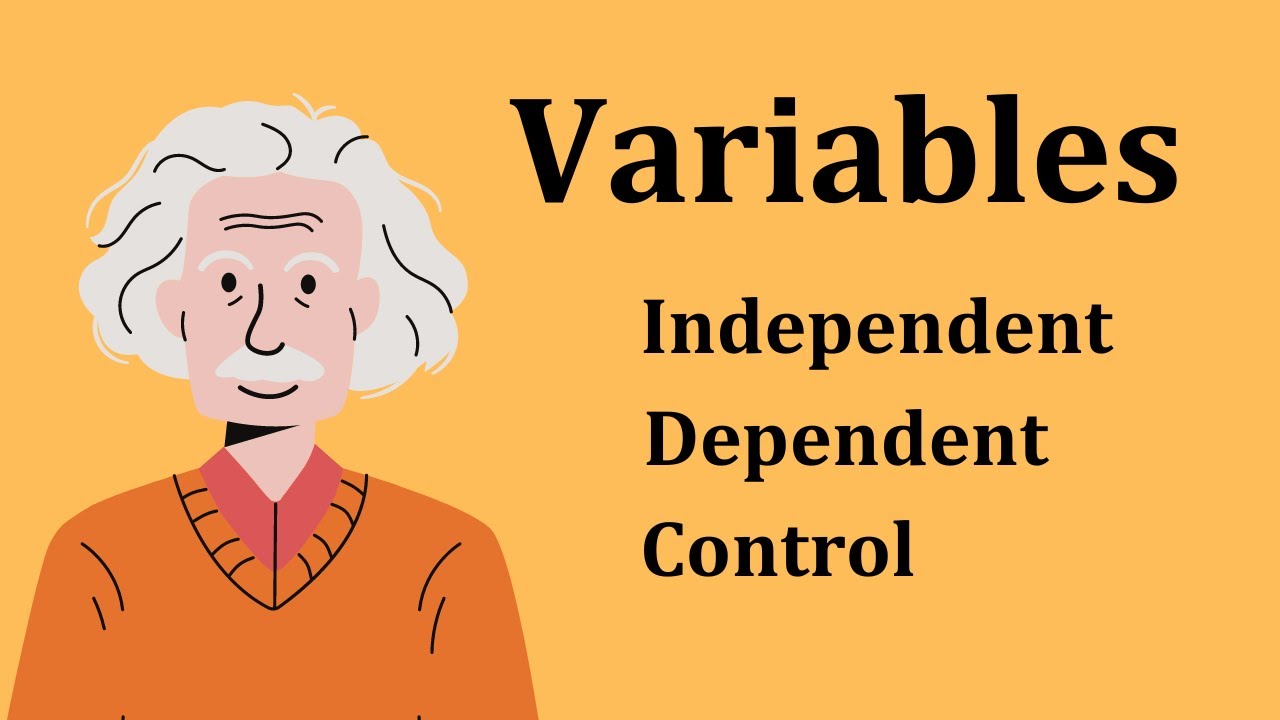
Image taken from the YouTube channel MooMooMath and Science , from the video titled Independent,Dependent, and Control Variables .
Unveiling the Power of Manipulated Variables in Research
In the realm of scientific inquiry, the pursuit of knowledge hinges on our ability to dissect, understand, and ultimately explain the relationships between different phenomena. At the heart of this endeavor lies the concept of manipulated variables, also known as independent variables.
These variables serve as the cornerstone of experimental research, offering a powerful lens through which we can explore cause-and-effect relationships.
Defining Manipulated Variables
A manipulated variable, or independent variable, is the factor that a researcher deliberately changes or alters in an experiment.
This manipulation is carried out to observe its effect on another variable, known as the dependent variable.
Essentially, it's the "cause" we are testing in a cause-and-effect scenario.
The Central Role in Experimental Research and Causality
Manipulated variables are the engine driving experimental research. By systematically changing the independent variable, researchers can observe and measure the resulting changes in the dependent variable.
This controlled manipulation allows us to infer, with a degree of confidence, whether a causal relationship exists between the two.
Establishing causality is a primary goal of scientific research, and manipulated variables are indispensable tools in achieving this aim.
The Importance of Proper Manipulation
The validity of any experimental results is directly tied to the way the independent variable is manipulated.
A poorly designed manipulation can lead to skewed results, making it difficult or impossible to draw meaningful conclusions.
For example, failing to control extraneous factors or introducing bias into the manipulation process can compromise the integrity of the experiment.
Therefore, careful planning, precise execution, and rigorous control are essential for ensuring that the manipulation of the independent variable yields valid and reliable data.
Scope of This Discussion
This discussion aims to provide a comprehensive understanding of manipulated variables and their role in scientific research.
We will explore the key concepts surrounding manipulated variables, including their definition, their importance in establishing causality, and the principles of proper manipulation.
By the end of this discussion, you should have a solid understanding of how to effectively use manipulated variables to design experiments and interpret results.
Causation vs. Correlation: Distinguishing Relationships Between Variables
Unveiling the Power of Manipulated Variables in Research
In the realm of scientific inquiry, the pursuit of knowledge hinges on our ability to dissect, understand, and ultimately explain the relationships between different phenomena. At the heart of this endeavor lies the concept of manipulated variables, also known as independent variables.
These variables are the levers we pull in experiments, the factors we deliberately change to observe their effects on other variables. This allows researchers to draw definitive conclusions on why changes occurred in a study.
However, navigating the world of research requires a keen understanding of the crucial difference between causation and correlation. While correlation may suggest a relationship between two variables, it doesn't automatically mean that one causes the other. Manipulated variables can help in a careful scientific process to discover the real reason behind changes, but only if used correctly.
This distinction is paramount for interpreting experimental results accurately and avoiding flawed conclusions.
Understanding Causation
At its core, causation implies a direct relationship where one event (the cause) leads to another event (the effect). Establishing causation requires demonstrating that a change in one variable directly results in a change in another.
This is more than just observing that two variables tend to move together. It necessitates evidence that the cause precedes the effect and that no other factors could explain the observed relationship.
Rigorous Requirements for Establishing Causation
Demonstrating causation is a rigorous process, often requiring adherence to a strict set of criteria. One commonly cited framework is the Bradford Hill criteria, which outlines nine aspects to consider when assessing evidence of a causal relationship. These include:
- Strength: The stronger the association between the variables, the more likely it is causal.
- Consistency: The association should be observed repeatedly in different settings and populations.
- Specificity: The cause should lead to a specific effect, rather than a wide range of outcomes.
- Temporality: The cause must precede the effect in time.
- Biological Gradient: A dose-response relationship should exist, where increasing the exposure to the cause leads to a greater effect.
- Plausibility: The relationship should be biologically plausible, fitting with current scientific understanding.
- Coherence: The causal interpretation should not conflict with other known facts about the disease.
- Experiment: Evidence from experimental studies strengthens the causal argument.
- Analogy: Similar relationships have been shown to be causal for other exposures and outcomes.
While these criteria provide a valuable framework, it's important to note that they are not absolute requirements. The weight given to each criterion depends on the specific context of the research question.
Examples of Causal Relationships
A classic example of a causal relationship is the link between smoking and lung cancer. Numerous studies have consistently demonstrated that smoking directly increases the risk of developing lung cancer.
The evidence supporting this causal link is strong, consistent, specific, and temporally aligned.
Another example is the relationship between exercise and cardiovascular health. Regular physical activity has been shown to improve various markers of cardiovascular health, such as blood pressure, cholesterol levels, and heart function. Again, the weight of evidence supports a causal link between exercise and improved cardiovascular outcomes.
Understanding Correlation
Correlation, on the other hand, simply indicates a statistical relationship between two or more variables. This means that the variables tend to move together, either in the same direction (positive correlation) or in opposite directions (negative correlation).
For example, ice cream sales and crime rates often exhibit a positive correlation – as ice cream sales increase, so does the crime rate. However, this doesn't mean that eating ice cream causes people to commit crimes, or vice versa.
Why Correlation Does Not Equal Causation
The critical point to remember is that correlation does not equal causation. Just because two variables are related doesn't mean that one causes the other. There are several reasons why a correlation might exist without a causal relationship:
- Reverse Causation: The apparent "effect" might actually be causing the apparent "cause."
- Third Variable: A third, unmeasured variable might be influencing both variables, creating the illusion of a relationship between them.
- Chance: The correlation could simply be due to random chance, especially in small sample sizes.
Examples of Correlated but Non-Causal Relationships
Returning to the ice cream and crime rate example, the correlation is likely due to a third variable: warm weather. During warmer months, people tend to buy more ice cream, and they also tend to spend more time outdoors, which creates more opportunities for crime.
Therefore, the relationship between ice cream sales and crime rate is spurious – it's not a direct causal link, but rather an indirect association driven by a common underlying factor.
Another classic example is the correlation between the number of storks nesting on roofs and the number of births in a region. While a statistical relationship may exist, it's highly unlikely that storks are actually delivering babies. This correlation is likely due to other factors, such as rural versus urban populations and environmental conditions.
Spurious Correlations and Confounding Factors
Spurious correlations are those that appear to be meaningful but are actually due to chance or the influence of a third variable. These correlations can be misleading and can lead to incorrect conclusions if not carefully examined.
Confounding factors are variables that are related to both the independent and dependent variables, obscuring the true relationship between them. Confounding factors can create the illusion of a causal relationship when none exists, or they can mask a true causal relationship.
Identifying and controlling for confounding factors is a critical step in research design.
Formulating and Testing Hypotheses: The Core of Scientific Inquiry
Building upon the crucial distinction between correlation and causation, we now turn our attention to the very engine of scientific discovery: the formulation and testing of hypotheses. A well-crafted hypothesis serves as a compass, guiding the experimental design and subsequent data analysis, ultimately leading to a deeper understanding of the natural world.
Understanding the Hypothesis
At its core, a hypothesis is a testable statement, a proposed explanation for a phenomenon. It is not a mere guess, but rather an educated prediction based on existing knowledge and observations.
The primary purpose of a hypothesis is to establish a clear relationship between the independent (manipulated) and dependent (measured) variables.
It essentially proposes how a change in the independent variable will influence the dependent variable. Without this clearly defined link, experimental results become difficult to interpret and may lack meaningful implications.
Crafting a Well-Formulated Hypothesis
A robust hypothesis possesses several key characteristics. It must be:
-
Testable: The hypothesis must be amenable to experimental investigation. It should be possible to design an experiment that could potentially provide evidence to either support or refute the hypothesis.
-
Falsifiable: The hypothesis must be capable of being proven wrong. If there is no conceivable way to disprove the hypothesis, it lacks scientific value.
-
Specific: The hypothesis should clearly define the variables involved and the nature of the relationship between them. Vague or ambiguous hypotheses lead to inconclusive results.
For example, instead of stating "Exercise affects health," a more well-formulated hypothesis might be: "Thirty minutes of daily moderate-intensity exercise will lead to a decrease in resting heart rate in sedentary adults."
This refined hypothesis is testable, falsifiable (resting heart rate might not decrease), and specific about the type and duration of exercise, the population being studied, and the outcome being measured.
Null and Alternative Hypotheses
In the realm of statistical hypothesis testing, we encounter two complementary hypotheses: the null hypothesis and the alternative hypothesis.
The null hypothesis (often denoted as H0) represents the statement of "no effect" or "no relationship" between the variables under investigation. It is the hypothesis that the researcher aims to disprove.
The alternative hypothesis (often denoted as H1 or Ha) represents the researcher's actual prediction – the statement that there is a relationship between the variables.
In our exercise example, the null hypothesis would be: "Thirty minutes of daily moderate-intensity exercise will not lead to a decrease in resting heart rate in sedentary adults." The alternative hypothesis, as stated earlier, would be: "Thirty minutes of daily moderate-intensity exercise will lead to a decrease in resting heart rate in sedentary adults."
Testing the Hypothesis Through Experimentation
Once a hypothesis is formulated, the next step is to design and conduct an experiment to test its validity.
This process involves:
-
Selecting an appropriate experimental design: Choosing a design that allows for the manipulation of the independent variable and the measurement of the dependent variable while controlling for extraneous factors.
-
Collecting data: Gathering relevant data through careful observation and measurement.
-
Analyzing the data: Applying statistical methods to determine whether the data provides sufficient evidence to reject the null hypothesis in favor of the alternative hypothesis.
-
Interpreting the results: Drawing conclusions based on the statistical analysis, considering the limitations of the study, and discussing the implications of the findings.
It is crucial to remember that failing to reject the null hypothesis does not necessarily prove it is true. It simply means that the data did not provide enough evidence to reject it. Further research may be needed to confirm or refute the null hypothesis. The ultimate goal of testing a hypothesis is to refine our understanding of the world, even when the results are not as expected.
Designing Effective Experiments: A Roadmap for Valid Results
Formulating a testable hypothesis is only the first step. The true power of the scientific method lies in its rigorous methodology, which demands a carefully constructed experimental design to translate those hypotheses into meaningful and reliable results. A poorly designed experiment, regardless of the brilliance of the initial idea, is likely to yield ambiguous or misleading data, ultimately undermining the entire research endeavor.
What is Experimental Design?
At its core, experimental design is the blueprint for conducting research. It's a structured approach that meticulously outlines every aspect of the experiment, from participant selection to data collection methods and analysis techniques. The primary aim of a sound experimental design is to minimize bias and maximize the likelihood of obtaining valid and reliable results that directly address the research question.
Types of Experimental Designs
The landscape of experimental designs is diverse, offering researchers a range of options tailored to specific research questions and constraints. Each type has its strengths and weaknesses, making the choice dependent on the specific context.
Randomized Controlled Trials (RCTs)
Often considered the gold standard, RCTs involve randomly assigning participants to either an experimental group, which receives the treatment, or a control group, which does not. Randomization is key in minimizing selection bias, ensuring that the groups are as similar as possible at the outset. This design is particularly powerful for establishing cause-and-effect relationships.
Within-Subjects Designs
In contrast to RCTs, within-subjects designs expose each participant to all conditions or treatments being tested. This approach eliminates individual differences as a source of variability, but it introduces the potential for order effects, such as learning or fatigue. Researchers must carefully consider these factors and employ techniques like counterbalancing to mitigate their impact.
Considerations for Choosing an Appropriate Design
Selecting the right experimental design is a critical decision, influenced by a multitude of factors. The nature of the research question, the availability of resources, ethical considerations, and the characteristics of the study population all play a role.
- Research Question: Is the goal to compare two groups or to assess the effects of multiple treatments on the same individuals?
- Resources: How many participants are available? What is the budget for the study?
- Ethical Concerns: Are there any potential risks to participants?
- Study Population: Are there any specific characteristics of the population that need to be considered?
A careful evaluation of these factors is essential for choosing the most appropriate and feasible design.
The Importance of Randomization
Randomization is a cornerstone of experimental design, particularly in RCTs. It's a process that ensures each participant has an equal chance of being assigned to any of the experimental groups.
This seemingly simple step has profound implications for the validity of the study. By minimizing selection bias, randomization helps to create comparable groups, allowing researchers to confidently attribute any observed differences to the treatment rather than pre-existing differences between the groups.
The Importance of Appropriate Sample Size
The size of the sample, or the number of participants in the study, is another critical determinant of the experiment's power to detect meaningful effects. A sample size that is too small may lack the statistical power to detect a real effect, leading to a false negative conclusion. Conversely, a sample size that is too large may detect statistically significant but practically insignificant effects, wasting resources and potentially exposing more participants to unnecessary risks.
Researchers must carefully calculate the appropriate sample size based on the expected effect size, the desired level of statistical power, and the acceptable risk of a false positive result. This often involves using statistical software and consulting with a statistician to ensure the study is adequately powered.
Ensuring Validity and Reliability: Strengthening the Integrity of Research
Designing Effective Experiments: A Roadmap for Valid Results Formulating a testable hypothesis is only the first step. The true power of the scientific method lies in its rigorous methodology, which demands a carefully constructed experimental design to translate those hypotheses into meaningful and reliable results. A poorly designed experiment, regardless of the brilliance of the initial hypothesis, can yield data that is misleading or, worse, completely useless.
The pursuit of knowledge hinges not only on asking the right questions, but also on the integrity of the methods used to answer them. Two pillars upholding this integrity are validity and reliability.
Without these cornerstones, research findings are vulnerable to misinterpretation, undermining the entire scientific process.
The Cornerstone of Validity: Measuring What Matters
At its core, validity addresses whether a study truly measures what it intends to measure. It's about the accuracy and truthfulness of the research findings.
Imagine trying to measure intelligence using a ruler – the tool is simply inappropriate. Similarly, a research study lacking validity is using an inappropriate instrument or approach, yielding results that may appear meaningful but are fundamentally flawed.
Internal Validity: Establishing Cause and Effect
Internal validity focuses on the extent to which a study establishes a genuine cause-and-effect relationship between the manipulated variable and the observed outcome. In other words, can we confidently say that the independent variable caused the change in the dependent variable, rather than some other extraneous factor?
A study with high internal validity carefully controls for confounding variables, minimizing the risk that alternative explanations might account for the results. Random assignment, blinding, and the use of control groups are essential strategies for bolstering internal validity.
Failure to adequately control for extraneous influences can lead to spurious conclusions, where the apparent effect of the independent variable is, in reality, attributable to something else entirely.
External Validity: Generalizing Beyond the Study
External validity concerns the generalizability of the research findings. To what extent can the results of a study be applied to other populations, settings, and times?
A study with high external validity produces results that are robust and applicable beyond the specific confines of the experiment.
Factors influencing external validity include the representativeness of the sample, the ecological validity of the experimental setting (i.e., how closely it resembles real-world conditions), and the consistency of the findings across different contexts.
Research lacking external validity may be of limited practical significance, as its findings may not translate to real-world situations.
Controlled Variables: Guardians of Validity
Controlled variables play a crucial role in enhancing validity. By holding constant factors that could potentially influence the dependent variable, researchers can isolate the effect of the independent variable and strengthen the causal inference.
Failing to control for relevant variables introduces noise into the data, making it difficult to discern the true relationship between the variables of interest.
For example, in a study investigating the effect of a new fertilizer on plant growth, it would be essential to control for factors such as sunlight exposure, watering frequency, and soil type. Otherwise, differences in plant growth could be attributed to these uncontrolled variables rather than solely to the fertilizer.
The Foundation of Reliability: Consistent and Dependable Results
While validity concerns the accuracy of the measurement, reliability focuses on its consistency and dependability. A reliable study produces similar results when repeated under the same conditions.
Think of a bathroom scale that gives you a different weight every time you step on it – that scale is unreliable. Similarly, a research study lacking reliability yields inconsistent results, making it difficult to draw firm conclusions.
Assessing Reliability: Methods for Ensuring Consistency
Several methods exist for assessing the reliability of research findings.
-
Test-retest reliability: This involves administering the same test or measure to the same group of participants on two separate occasions and examining the correlation between the scores. A high correlation indicates good test-retest reliability.
-
Inter-rater reliability: This is used when data are collected through observation or judgment. It involves having multiple raters independently assess the same data and examining the degree of agreement between their ratings. High agreement indicates good inter-rater reliability.
Replication: The Ultimate Test of Reliability
One of the most powerful ways to confirm the reliability of research findings is through replication. If other researchers can independently replicate the study and obtain similar results, this provides strong evidence that the original findings are robust and not due to chance or error.
The replication crisis in several scientific fields highlights the importance of replication in ensuring the trustworthiness of research. A failure to replicate original findings raises serious questions about the validity and reliability of the initial study.
In conclusion, validity and reliability are indispensable for producing credible and trustworthy research. They are not merely technical details but fundamental principles that underpin the scientific endeavor. By carefully attending to these aspects of research design, researchers can strengthen the integrity of their findings and contribute meaningfully to the advancement of knowledge.
Statistical Significance: Determining the Probability of Results
[Ensuring Validity and Reliability: Strengthening the Integrity of Research Designing Effective Experiments: A Roadmap for Valid Results Formulating a testable hypothesis is only the first step. The true power of the scientific method lies in its rigorous methodology, which demands a carefully constructed experimental design to translate those hypotheses into concrete, measurable outcomes. But even the most meticulous experiment yields results that must be carefully interpreted, and a key tool in this interpretation is the concept of statistical significance.]
Statistical significance is a cornerstone of scientific research, but it's also one of the most frequently misunderstood. It provides a framework for assessing the probability that observed results are due to a real effect, rather than random chance. However, it's crucial to understand its limitations and to avoid over-interpreting its meaning.
Defining Statistical Significance
At its core, statistical significance indicates the likelihood that the relationship observed between variables in a sample also exists in the broader population. It's a probabilistic statement, not an absolute declaration of truth.
A result is considered statistically significant if the probability of observing it by chance alone is below a pre-determined threshold, often set at 5% (p < 0.05). This threshold, known as the alpha level, represents the maximum risk a researcher is willing to take of concluding there is an effect when, in reality, there isn't one (a Type I error).
The Role of P-values
The p-value is the central metric in determining statistical significance. It represents the probability of obtaining results as extreme as, or more extreme than, those observed, assuming that the null hypothesis is true.
The null hypothesis posits that there is no real effect or relationship between the variables under investigation. A small p-value (e.g., p < 0.05) provides evidence against the null hypothesis, suggesting that the observed results are unlikely to have occurred by chance.
However, it’s vital to remember that the p-value doesn’t tell us the size or importance of the effect, only the probability that it isn't due to random variation.
Limitations and Misinterpretations
While statistical significance is a valuable tool, it's essential to recognize its inherent limitations:
-
Statistical significance does not equal practical significance. A statistically significant result may be too small to have any real-world relevance. The size of the effect, often measured by effect size statistics, should also be considered.
-
Statistical significance does not prove causation. Correlation does not equal causation, and a statistically significant association between variables does not automatically imply that one variable causes the other. Confounding variables, reverse causality, and other factors can influence the observed relationship.
-
P-values are easily misinterpreted. The p-value is not the probability that the null hypothesis is true, nor is it the probability that the alternative hypothesis is false. It's the probability of observing the data if the null hypothesis were true.
-
Over-reliance on p<0.05 can lead to publication bias. Studies with statistically significant results are more likely to be published, which can create a distorted view of the evidence.
Confidence Intervals: Providing a Range of Plausible Values
Confidence intervals provide a range of values within which the true population parameter is likely to fall. Instead of providing a single point estimate, confidence intervals offer a more nuanced view of the uncertainty surrounding the results.
A 95% confidence interval, for example, means that if the study were repeated many times, 95% of the resulting intervals would contain the true population parameter. Confidence intervals provide valuable information about the precision of the estimate and the potential range of values that are consistent with the data.
Video: Manipulated Variable: Definition & Examples
FAQs about Manipulated Variables
What happens to the manipulated variable during an experiment?
The manipulated variable, also known as the independent variable, is deliberately changed or controlled by the researcher. This change is done to see what effect it has on another variable. The researcher actively adjusts the levels of the manipulated variable throughout the experiment.
How does the manipulated variable relate to the responding variable?
The manipulated variable is the presumed cause, and its change is expected to impact the responding variable (also known as the dependent variable). Scientists observe and measure the responding variable to see if it is affected by the alterations to the manipulated variable. Essentially, the manipulated variable influences the responding variable.
Can a study have more than one manipulated variable?
Yes, a study can have multiple manipulated variables. Researchers might want to examine how several factors, or a combination of factors, influence the responding variable. The presence of multiple manipulated variables can increase the complexity of the experiment.
What is an example of a manipulated variable in a plant growth experiment?
In a plant growth experiment, the amount of fertilizer given to different groups of plants could be the manipulated variable. The researcher controls how much fertilizer each plant receives. The plant's height or weight, measured after a certain period, would be the responding variable, showing the effect of the different fertilizer amounts (the manipulated variable).
So, there you have it! Understanding the manipulated variable is key to unlocking the secrets behind how experiments work. Now that you know what it is and how it differs from other variables, you're well on your way to designing your own experiments or understanding the science behind the world around you. Happy experimenting!