ANOVA Table Explained: Unlock Statistical Insights Now!
The anova table, a cornerstone of statistical analysis, presents a structured format for interpreting variance within datasets. Understanding its components allows for robust conclusions regarding the significance of group differences. Ronald Fisher, a pioneer in statistical methodology, laid much of the groundwork for the techniques used in its construction. SPSS (Statistical Package for the Social Sciences), a widely used statistical software package, provides tools for generating and interpreting the anova table. These interpretations are critical for decision-making in various fields, including in research and quality control.
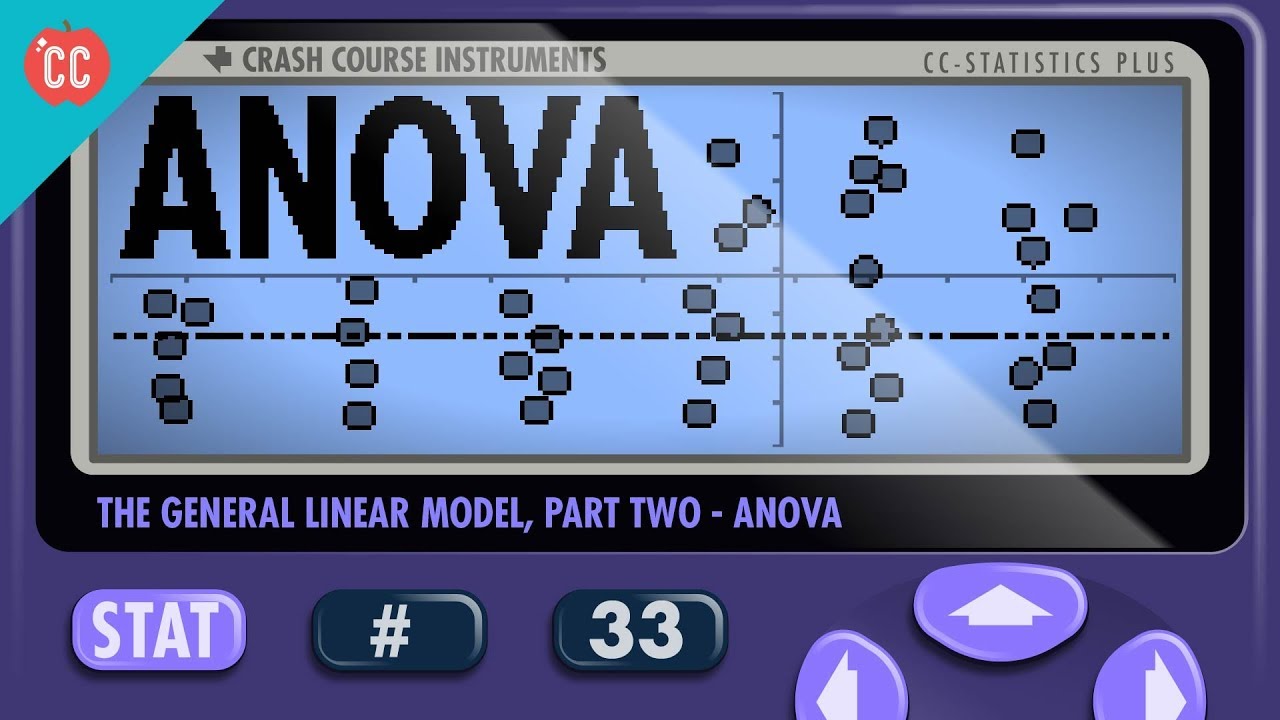
Image taken from the YouTube channel CrashCourse , from the video titled ANOVA: Crash Course Statistics #33 .
In the realm of statistical analysis, few tools possess the versatility and analytical power of ANOVA, or Analysis of Variance. ANOVA serves as a cornerstone for researchers and data analysts seeking to understand the differences between group means within a dataset. It’s a method used to tease out meaningful variations and patterns.
The ANOVA table, in particular, acts as a central point of reference. It is the summary of the entire test.
Understanding the ANOVA table is critical for anyone aiming to make informed, data-driven decisions. Without the ability to properly read an ANOVA table, the conclusions are likely to be flawed.
The goal of this article is to provide a comprehensive, reader-friendly explanation of ANOVA tables, dissecting their components and clarifying their interpretation. From source of variation to p-value, we’ll explore each element to provide readers with a robust foundation for understanding and applying ANOVA in their own work.
ANOVA: A Brief Overview
ANOVA allows us to compare the means of two or more groups.
Unlike simpler tests, like the t-test (which is best suited for comparing only two groups), ANOVA provides a framework for handling more complex experimental designs. These designs are those where multiple groups are being compared simultaneously.
This makes it invaluable in fields ranging from medicine and psychology to engineering and marketing. In each, the analysis of variance is invaluable.
The Importance of Deciphering ANOVA Tables
At its core, the ANOVA table summarizes the results of the ANOVA test.
It presents a structured breakdown of the variance within a dataset. Understanding how to interpret each element within the table is critical. It allows researchers to determine whether observed differences between groups are statistically significant or simply due to random chance.
The ANOVA table helps to extract meaningful insights from complex data and draw sound conclusions based on statistical evidence.
It goes beyond simply stating whether a difference exists, but rather quantifies the significance of that difference.
Thesis Statement: A Comprehensive Guide
This article aims to equip readers with the knowledge and skills necessary to confidently interpret ANOVA tables.
We will explore each component, from the sums of squares to the F-statistic and p-value. The significance of these factors will be explored and explained.
Through clear explanations and practical examples, we will unravel the complexities of ANOVA. This will allow readers to effectively apply this powerful statistical tool in their own research and analysis.
In the realm of statistical analysis, few tools possess the versatility and analytical power of ANOVA, or Analysis of Variance. ANOVA serves as a cornerstone for researchers and data analysts seeking to understand the differences between group means within a dataset. It’s a method used to tease out meaningful variations and patterns.
The ANOVA table, in particular, acts as a central point of reference. It is the summary of the entire test. Understanding the ANOVA table is critical for anyone aiming to make informed, data-driven decisions. Without the ability to properly read an ANOVA table, the conclusions are likely to be flawed.
The goal of this article is to provide a comprehensive, reader-friendly explanation of ANOVA tables, dissecting their components and clarifying their interpretation. From source of variation to p-value, we’ll explore each element to provide readers with a robust foundation for understanding and applying ANOVA in their own work.
ANOVA allows us to compare the means of two or more groups. Unlike simpler tests, like the t-test (which is best suited for comparing only two groups), ANOVA provides a framework for handling more complex experimental designs. These designs are those where multiple groups are being compared simultaneously.
This makes it invaluable in fields ranging from medicine and psychology to engineering and marketing. In each, the analysis of variance is invaluable. At its core, the ANOVA table summarizes the results of the ANOVA test.
It presents a structured breakdown of the data. But before we dive into the anatomy of the ANOVA table itself, it’s important to set the stage.
Let’s take a closer look at the fundamental principles that underpin ANOVA. This will help you understand when to use it and why it’s such a powerful statistical tool.
ANOVA Fundamentals: A Statistical Compass
At its heart, ANOVA (Analysis of Variance) is a statistical test that determines whether there are statistically significant differences between the means of two or more independent groups.
It achieves this by partitioning the total variance observed in the data into different sources, allowing us to assess the relative contribution of each source to the overall variability. This partitioning is key to understanding group differences.
Decoding the Definition
ANOVA is not simply about comparing means; it’s about analyzing variance. It examines how much the groups differ from each other relative to the variability within each group.
This distinction is crucial because even if the means are different, the difference might not be statistically significant if the within-group variability is large. Essentially, ANOVA determines if the differences we see are real or due to chance.
When to Employ ANOVA
ANOVA shines in scenarios where you need to compare the means of three or more groups. This is common in experimental research, where you might have a control group and multiple treatment groups.
Some common examples include:
- Comparing the effectiveness of several different drugs.
- Analyzing customer satisfaction scores across different product lines.
- Evaluating the yield of crops under different fertilizer treatments.
ANOVA is most appropriate when the data meets certain assumptions. These include:
- Normality: The data within each group should be approximately normally distributed.
- Homogeneity of Variance: The variance within each group should be roughly equal.
- Independence: Observations should be independent of each other.
We’ll delve into these assumptions and how to address violations later. But for now, it’s important to keep them in mind when deciding if ANOVA is the right tool.
ANOVA vs. T-tests: Handling Multiple Groups
When comparing the means of only two groups, a t-test is often the go-to choice. But when dealing with three or more groups, using multiple t-tests is not recommended.
Here's why:
- Increased Risk of Type I Error: Each t-test carries a risk of falsely rejecting the null hypothesis (Type I error). Performing multiple t-tests increases the overall probability of making at least one Type I error.
- ANOVA Controls for Experiment-wise Error: ANOVA, on the other hand, controls for the experiment-wise error rate, ensuring that the overall probability of making a Type I error remains at the desired level (typically 0.05).
In essence, ANOVA provides a more robust and reliable way to compare multiple group means. It provides a bird's-eye view of the data, preventing the inflation of error rates associated with multiple pairwise comparisons.
Anatomy of an ANOVA Table: Decoding the Elements
But before we dive into the anatomy of the ANOVA table, it's helpful to recognize its comprehensive and organized approach. The table is not merely a collection of numbers. Instead, it's a structured summary of the variance within your data. This structured format enables a clear understanding of how different sources of variation contribute to the overall outcome. Now, let's carefully dissect each component of this statistical tool.
Source of Variation: Unpacking the Origins of Variance
The Source of Variation column is the starting point. It identifies the different sources contributing to the total variance observed in the data. Typically, you'll encounter two primary sources: Between-group variation and Within-group variation.
Between-group variation, also known as treatment or factor effect, reflects the variability in the data that is due to the differences between the groups being compared. It assesses whether the group means are significantly different from each other. A large between-group variation suggests that the independent variable has a significant impact on the dependent variable.
Within-group variation, also known as error or residual variation, captures the variability within each group. It represents the random, unexplained variation that isn't attributable to the differences between groups. This could be due to individual differences, measurement errors, or other factors not controlled in the study. A smaller within-group variation suggests that the data within each group are relatively consistent.
Sum of Squares (SS): Quantifying Variation
The Sum of Squares (SS) is a measure of the total variability for each source of variation. It quantifies the spread or dispersion of data points around their respective means. In simpler terms, it reflects the total amount of variation observed for each source.
The formula for calculating SS varies depending on the source of variation. The Sum of Squares Between (SSB) quantifies the variation between the group means and the overall mean, while the Sum of Squares Within (SSW) quantifies the variation within each group.
A higher SS value indicates greater variability. In the context of ANOVA, comparing the SS values between groups and within groups helps determine whether the variability between groups is significantly larger than the variability within groups. This difference suggests that the independent variable has a significant effect on the dependent variable.
Degrees of Freedom (DF): Allocating Variance
Degrees of Freedom (DF) refer to the number of independent pieces of information available to estimate a parameter. In other words, it represents the number of values in the final calculation of a statistic that are free to vary. Understanding degrees of freedom is crucial for correctly interpreting the ANOVA table.
The calculation of DF differs for between-group and within-group variation. For between-group DF, it is calculated as the number of groups minus one (k - 1), where k is the number of groups. For within-group DF, it is calculated as the total number of observations minus the number of groups (N - k), where N is the total sample size.
DF influences the shape of the F-distribution, which is used to determine the p-value. A higher DF generally leads to a more sensitive test, increasing the likelihood of detecting a statistically significant difference if one exists.
Mean Square (MS): Averaging the Variation
The Mean Square (MS) is calculated by dividing the Sum of Squares (SS) by its corresponding Degrees of Freedom (DF). It represents the average variability for each source of variation. MS provides a standardized measure of variance, making it easier to compare the variability between different sources.
The Mean Square Between (MSB) is calculated by dividing SSB by its corresponding DF (k - 1), while the Mean Square Within (MSW) is calculated by dividing SSW by its corresponding DF (N - k).
MS values are essential for calculating the F-statistic. By comparing MSB to MSW, we can determine whether the variance between groups is significantly larger than the variance within groups.
F-statistic: Testing the Hypothesis
The F-statistic is the core of the ANOVA test. It is calculated by dividing the Mean Square Between (MSB) by the Mean Square Within (MSW). In essence, it's a ratio that compares the variance between groups to the variance within groups.
A large F-statistic indicates that the variance between groups is substantially larger than the variance within groups. This suggests that the group means are significantly different from each other. Conversely, a small F-statistic suggests that the variance between groups is similar to the variance within groups, indicating no significant difference between the group means.
The F-statistic is used to test the null hypothesis, which states that there are no significant differences between the group means. By comparing the calculated F-statistic to a critical value from the F-distribution, we can determine whether to reject or fail to reject the null hypothesis.
P-value: Determining Statistical Significance
The P-value represents the probability of observing an F-statistic as extreme as, or more extreme than, the one calculated from the data, assuming that the null hypothesis is true. It provides a measure of the evidence against the null hypothesis. In simpler terms, it tells us how likely it is that the observed results occurred by chance.
A small p-value (typically less than 0.05) indicates strong evidence against the null hypothesis. It suggests that the observed differences between the group means are statistically significant and not due to random chance. Therefore, we would reject the null hypothesis and conclude that there is a significant effect of the independent variable on the dependent variable.
A large p-value (typically greater than 0.05) indicates weak evidence against the null hypothesis. It suggests that the observed differences between the group means could be due to random chance. In this case, we would fail to reject the null hypothesis and conclude that there is no significant effect of the independent variable on the dependent variable.
The Sum of Squares and Degrees of Freedom give context to the variation within the data, and we can use that to calculate the Mean Square. But it's the F-statistic and the P-value that truly tell the story, offering insights into the statistical significance of our findings.
F-statistic and P-value: Interpreting the Statistical Story
The F-statistic and P-value are at the heart of hypothesis testing in ANOVA, providing the evidence needed to either reject or fail to reject the null hypothesis. Understanding their individual roles, their relationship to each other, and their connection to the degrees of freedom is critical for correctly interpreting ANOVA results. Beyond this, awareness of potential errors (Type I and Type II) ensures a nuanced and cautious approach to statistical inference.
Deciphering the F-statistic: Testing the Null Hypothesis
The F-statistic is a ratio of two variances: the variance between groups (Mean Square Between) and the variance within groups (Mean Square Within).
F = MSBetween / MSWithin
A large F-statistic suggests that the variance between groups is considerably greater than the variance within groups, indicating a potential difference between the group means.
In essence, the F-statistic quantifies how much of the total variance can be attributed to the treatment effect (the independent variable) compared to random error.
To test the null hypothesis (that there is no significant difference between group means), we compare our calculated F-statistic to a critical value from the F-distribution. This critical value depends on the degrees of freedom associated with the between-group and within-group variation, and the chosen significance level (alpha).
If the calculated F-statistic exceeds the critical value, we reject the null hypothesis, concluding that there is statistically significant evidence of a difference between at least two of the group means.
Unveiling the P-value: Assessing Statistical Significance
The P-value is the probability of observing a test statistic as extreme as, or more extreme than, the one calculated from the sample data, assuming the null hypothesis is true.
In simpler terms, it tells us how likely we are to see the observed results if there is actually no effect.
A small P-value (typically less than the significance level, α, which is often set at 0.05) indicates strong evidence against the null hypothesis. This suggests that the observed results are unlikely to have occurred by chance alone, and we reject the null hypothesis.
Conversely, a large P-value (greater than α) suggests that the observed results are reasonably likely to have occurred by chance, and we fail to reject the null hypothesis. This does not mean that the null hypothesis is true, only that we do not have sufficient evidence to reject it.
The P-value provides a direct measure of the strength of evidence against the null hypothesis, allowing researchers to make informed decisions about the statistical significance of their findings.
The Interplay: F-statistic, P-value, and Degrees of Freedom
The F-statistic, P-value, and degrees of freedom are intrinsically linked in ANOVA.
The F-statistic, along with the degrees of freedom, is used to calculate the P-value. The degrees of freedom influence the shape of the F-distribution, which in turn affects the P-value. A higher F-statistic, coupled with appropriate degrees of freedom, results in a smaller P-value, increasing the likelihood of rejecting the null hypothesis.
Essentially, the degrees of freedom act as a modifier, adjusting the interpretation of the F-statistic based on the sample size and the number of groups being compared.
Understanding this interplay is essential for accurate interpretation. A high F-statistic alone is not enough to declare statistical significance. The corresponding P-value, which takes into account the degrees of freedom, must also be sufficiently small.
Navigating Uncertainty: Type I and Type II Errors in ANOVA
In hypothesis testing, there is always a risk of making an incorrect decision.
A Type I error (false positive) occurs when we reject the null hypothesis when it is actually true. In the context of ANOVA, this means concluding that there is a significant difference between group means when, in reality, there is no difference. The probability of committing a Type I error is equal to the significance level (α).
A Type II error (false negative) occurs when we fail to reject the null hypothesis when it is actually false. In ANOVA, this means failing to detect a significant difference between group means when a real difference exists. The probability of committing a Type II error is denoted by β, and the power of the test (the probability of correctly rejecting a false null hypothesis) is 1 - β.
Several factors influence the likelihood of committing these errors, including the sample size, the magnitude of the effect, and the chosen significance level.
Increasing the sample size and/or the significance level can reduce the risk of a Type II error, but it increases the risk of a Type I error.
Researchers must carefully consider the consequences of each type of error when designing their studies and interpreting their results, balancing the risk of false positives and false negatives to draw meaningful and accurate conclusions.
The Sum of Squares and Degrees of Freedom give context to the variation within the data, and we can use that to calculate the Mean Square. But it's the F-statistic and P-value that truly tell the story, offering insights into the statistical significance of our findings.
ANOVA in Action: Real-World Examples
Statistical knowledge can often feel abstract until it's applied to tangible problems. Let's explore how ANOVA tables are utilized in real-world research scenarios, demonstrating how to interpret results and connect them back to the initial research questions.
Scenario 1: Comparing Teaching Methods
Imagine a study comparing the effectiveness of three different teaching methods on student test scores: traditional lecture, online modules, and collaborative projects. The researchers collect data on student performance after a semester of instruction using each method.
An ANOVA table would be generated to analyze the differences in mean test scores across the three groups.
The "Source of Variation" would include "Teaching Method" (between-group variation) and "Error" (within-group variation).
The F-statistic would test the null hypothesis that there is no significant difference in mean test scores among the three teaching methods.
The P-value would indicate the probability of observing the obtained results (or more extreme results) if the null hypothesis were true.
If the p-value is below a predetermined significance level (e.g., 0.05), the researchers would reject the null hypothesis and conclude that there is a statistically significant difference in the effectiveness of the teaching methods.
But here's where interpretation becomes critical. The ANOVA table only tells us that there's a difference, not where the difference lies. To pinpoint which teaching method(s) differ significantly from each other, post-hoc tests (e.g., Tukey's HSD) would be required.
Scenario 2: Analyzing Drug Effectiveness
Consider a pharmaceutical company investigating the effectiveness of a new drug in reducing blood pressure. Patients are randomly assigned to one of four groups: a placebo group, a low-dose group, a medium-dose group, and a high-dose group. Blood pressure measurements are taken after several weeks of treatment.
An ANOVA table would be used to determine if there are significant differences in mean blood pressure reduction among the four groups.
A significant F-statistic and a low p-value would suggest that the drug has a statistically significant effect on blood pressure.
However, similar to the teaching method example, further analysis is needed to determine which dosage levels are most effective and whether there are significant differences between specific dosage groups. Again, post-hoc tests are crucial.
Interpreting Results in Context
In both scenarios, it's vital to interpret the ANOVA results within the specific context of the research question. Statistical significance doesn't automatically equate to practical significance.
A small p-value might indicate a statistically significant difference, but the actual magnitude of the difference might be small and clinically irrelevant.
Researchers must consider the effect size, confidence intervals, and the practical implications of their findings.
The Connection to Regression
ANOVA and regression are closely related. In fact, ANOVA can be considered a special case of regression. When the independent variable in a regression model is categorical (e.g., teaching method, drug dosage), the regression analysis is equivalent to ANOVA.
Regression models can provide additional insights into the relationship between the independent and dependent variables, such as the direction and magnitude of the effect.
For example, in the teaching method scenario, a regression model could quantify the average difference in test scores between each teaching method and a baseline method.
Moreover, regression models can accommodate multiple independent variables, allowing researchers to examine the effects of several factors simultaneously. The ANOVA table in a regression context partitions the variance explained by the model from the unexplained variance. This allows assessment of how well the model fits the data.
The Sum of Squares and Degrees of Freedom give context to the variation within the data, and we can use that to calculate the Mean Square. But it's the F-statistic and P-value that truly tell the story, offering insights into the statistical significance of our findings.
Statistical tests like ANOVA are powerful tools, but their reliability hinges on certain underlying principles. Ignoring these foundational assumptions can lead to inaccurate conclusions, undermining the entire analysis. Let's examine these assumptions and how to address them when they are not met.
Assumptions and Alternatives: Navigating ANOVA's Boundaries
The Pillars of ANOVA: Key Assumptions
ANOVA, like many statistical tests, rests on several key assumptions about the data being analyzed. These assumptions ensure the validity and reliability of the results. The primary assumptions are:
-
Normality: The data within each group being compared should be approximately normally distributed. This means the data should follow a bell-shaped curve.
-
Homogeneity of Variance (Homoscedasticity): The variance (spread) of the data should be roughly equal across all groups.
-
Independence: The observations within each group should be independent of one another. Meaning one data point does not influence another.
These assumptions aren't arbitrary rules; they are the bedrock upon which ANOVA's calculations are built.
Violating these assumptions can lead to inflated Type I error rates (false positives) or decreased statistical power.
Testing the Waters: Assessing Assumption Validity
Before blindly accepting the results of an ANOVA, it's crucial to assess whether these assumptions hold true for your data. Several methods exist for testing these assumptions:
-
Normality Tests: Shapiro-Wilk, Kolmogorov-Smirnov, and Anderson-Darling tests can formally assess normality.
Visual inspection of histograms and Q-Q plots can also provide insights into the distribution of the data.
-
Homogeneity of Variance Tests: Levene's test and Bartlett's test are commonly used to assess the equality of variances across groups.
-
Independence Assessment: Independence is often assessed based on the study design and data collection procedures.
Random sampling and careful control of experimental conditions are essential for ensuring independence.
When Assumptions Crumble: Remedies for Violations
What happens when your data violates one or more of ANOVA's assumptions? The good news is that all is not lost. Several remedies can be employed:
-
Data Transformations: Applying mathematical transformations to the data (e.g., logarithmic, square root, inverse) can sometimes improve normality or homogeneity of variance.
Box-Cox transformations can be particularly useful for identifying the optimal transformation.
-
Non-Parametric Alternatives: Non-parametric tests, such as the Kruskal-Wallis test (for comparing multiple groups), do not rely on the same assumptions as ANOVA.
These tests are often more robust when dealing with non-normal data or unequal variances.
However, they may have less statistical power than ANOVA when assumptions are met.
Post-Hoc Tests: Unpacking the Differences
Even if the overall ANOVA indicates a significant difference between groups, it doesn't tell you which specific groups differ from each other. This is where post-hoc tests come in.
Post-hoc tests are conducted after a significant ANOVA result to perform pairwise comparisons between group means.
Common post-hoc tests include:
- Tukey's HSD (Honestly Significant Difference)
- Bonferroni correction
- Scheffé's method
- Dunnett's test (for comparing multiple groups to a control group)
The choice of post-hoc test depends on the specific research question and the characteristics of the data. Each test controls for Type I error in different ways.
A Nod to the Pioneer: Ronald Fisher and His Legacy
No discussion of ANOVA would be complete without acknowledging the contributions of Sir Ronald Fisher, a towering figure in the history of statistics.
Fisher developed ANOVA in the 1920s while working at an agricultural research station. His work revolutionized statistical analysis and laid the foundation for modern experimental design.
Fisher's insights into variance, hypothesis testing, and the importance of randomization continue to shape the field of statistics today.
His work is foundational to our understanding of how to draw meaningful conclusions from data.
The Sum of Squares and Degrees of Freedom give context to the variation within the data, and we can use that to calculate the Mean Square. But it's the F-statistic and P-value that truly tell the story, offering insights into the statistical significance of our findings.
Statistical tests like ANOVA are powerful tools, but their reliability hinges on certain underlying principles. Ignoring these foundational assumptions can lead to inaccurate conclusions, undermining the entire analysis. Let's examine these assumptions and how to address them when they are not met.
ANOVA with Software: R, SPSS, and Beyond
While understanding the underlying calculations of ANOVA is crucial, statistical software packages streamline the process, allowing researchers to focus on interpretation and analysis. Programs like R and SPSS are industry standards, providing user-friendly interfaces and powerful analytical capabilities to generate and interpret ANOVA tables. Let’s explore how these tools can be leveraged.
Generating ANOVA Tables in R
R, a free and open-source statistical computing environment, offers extensive functionality for ANOVA through various packages. The base R installation includes the aov()
function, which is commonly used to perform ANOVA tests.
To illustrate, consider a scenario where we want to compare the effect of three different fertilizers (A, B, and C) on plant growth. We can create a data frame in R and then use the aov()
function to perform the ANOVA.
# Sample data
fertilizer <- factor(rep(c("A", "B", "C"), each=10))
growth <- c(rnorm(10, 10, 2), rnorm(10, 12, 2), rnorm(10, 14, 2))
data <- data.frame(fertilizer, growth)
# Perform ANOVA
anovamodel <- aov(growth ~ fertilizer, data=data)
summary(anovamodel)
The summary(anova_model)
command displays the ANOVA table. This table provides the degrees of freedom, sum of squares, mean squares, F-statistic, and p-value, enabling you to assess the statistical significance of the fertilizer effect on plant growth.
The power of R lies in its flexibility and the vast number of packages available for advanced analyses. For instance, the emmeans
package can be used for post-hoc tests, while the ggplot2
package can create compelling visualizations of the data.
SPSS: A User-Friendly Approach to ANOVA
SPSS (Statistical Package for the Social Sciences) is a commercial statistical software known for its user-friendly graphical interface. Performing ANOVA in SPSS involves navigating through menus and dialog boxes, making it accessible to users with varying levels of statistical expertise.
To perform ANOVA in SPSS, you would typically import your data and then go to "Analyze" -> "Compare Means" -> "One-Way ANOVA". Specify your dependent variable (e.g., plant growth) and independent variable (e.g., fertilizer type). SPSS generates an ANOVA table similar to R, providing the same critical statistics for interpretation.
One of SPSS's strengths is its ease of use for conducting post-hoc tests. After running the ANOVA, you can select various post-hoc tests (e.g., Tukey's HSD, Bonferroni) to perform pairwise comparisons between group means. SPSS also offers a range of options for visualizing the data, such as boxplots and bar charts, which can enhance the interpretation of results.
SPSS is a popular choice for researchers who value a straightforward, menu-driven approach to statistical analysis.
Beyond R and SPSS: Other Notable Software
While R and SPSS are widely used, other statistical software packages offer ANOVA capabilities. These include:
- SAS (Statistical Analysis System): A comprehensive statistical software suite often used in business and industry.
- Stata: A statistical software popular in economics and epidemiology.
- JMP: A visual data analysis software developed by SAS Institute.
Each of these software packages offers unique strengths and caters to different user preferences. The choice of software often depends on factors such as cost, ease of use, and specific analytical needs.
Interpreting Software-Generated ANOVA Tables
Regardless of the software used, the interpretation of the ANOVA table remains consistent. The F-statistic and p-value are the key elements for determining statistical significance.
A small p-value (typically less than 0.05) indicates strong evidence against the null hypothesis, suggesting that there is a significant difference between the group means. Conversely, a large p-value suggests that there is no significant difference.
The degrees of freedom are important for understanding the F-statistic, as the F-distribution depends on the degrees of freedom for both the numerator (between-group variance) and the denominator (within-group variance).
Emphasizing Practical Application
The real value of using software for ANOVA lies in its ability to quickly and accurately perform complex calculations, allowing researchers to focus on the broader context of their research. By understanding the outputs generated by these tools, researchers can draw meaningful conclusions and make data-driven decisions.
By combining a solid understanding of ANOVA principles with proficiency in statistical software, analysts can unlock valuable insights from their data.
Video: ANOVA Table Explained: Unlock Statistical Insights Now!
ANOVA Table Explained: FAQs
Want a clearer understanding of ANOVA tables? Here are some frequently asked questions to help.
What exactly does an ANOVA table tell you?
An ANOVA table summarizes the results of an Analysis of Variance (ANOVA) test. It displays key values like degrees of freedom, sums of squares, mean squares, the F-statistic, and the p-value. These values allow you to determine if there are statistically significant differences between the means of two or more groups.
What is the F-statistic in an ANOVA table, and why is it important?
The F-statistic is a ratio of the variance between groups to the variance within groups. A larger F-statistic indicates greater variability between the group means relative to the variability within the groups. You compare it to a critical value to decide statistical significance.
How do I interpret the p-value in an ANOVA table?
The p-value represents the probability of observing the obtained results (or more extreme results) if there is no actual difference between the group means. A small p-value (typically less than 0.05) suggests strong evidence against the null hypothesis, indicating a statistically significant difference. Therefore you would reject the null hypothesis.
What are "degrees of freedom" in the context of an ANOVA table?
Degrees of freedom (df) represent the number of independent pieces of information used to calculate a statistic. The ANOVA table includes df for the groups (between-groups df) and the error (within-groups df). These values are crucial for determining the critical value when testing the null hypothesis regarding the ANOVA table.