AI's Ethical Minefield: What Are the Implications?
Artificial intelligence, with its transformative potential, presents complex challenges regarding what are ethical implications. The Partnership on AI, an organization dedicated to responsible AI development, emphasizes the importance of addressing biases ingrained within algorithms. Consequently, developers and policymakers alike must consider the potential for algorithmic bias to perpetuate societal inequalities. These issues are further complicated by the inherent limitations of explainable AI (XAI) in complex models, making it difficult to fully understand the decision-making processes and, thereby, assess what are ethical implications. Examining what are ethical implications is vital.
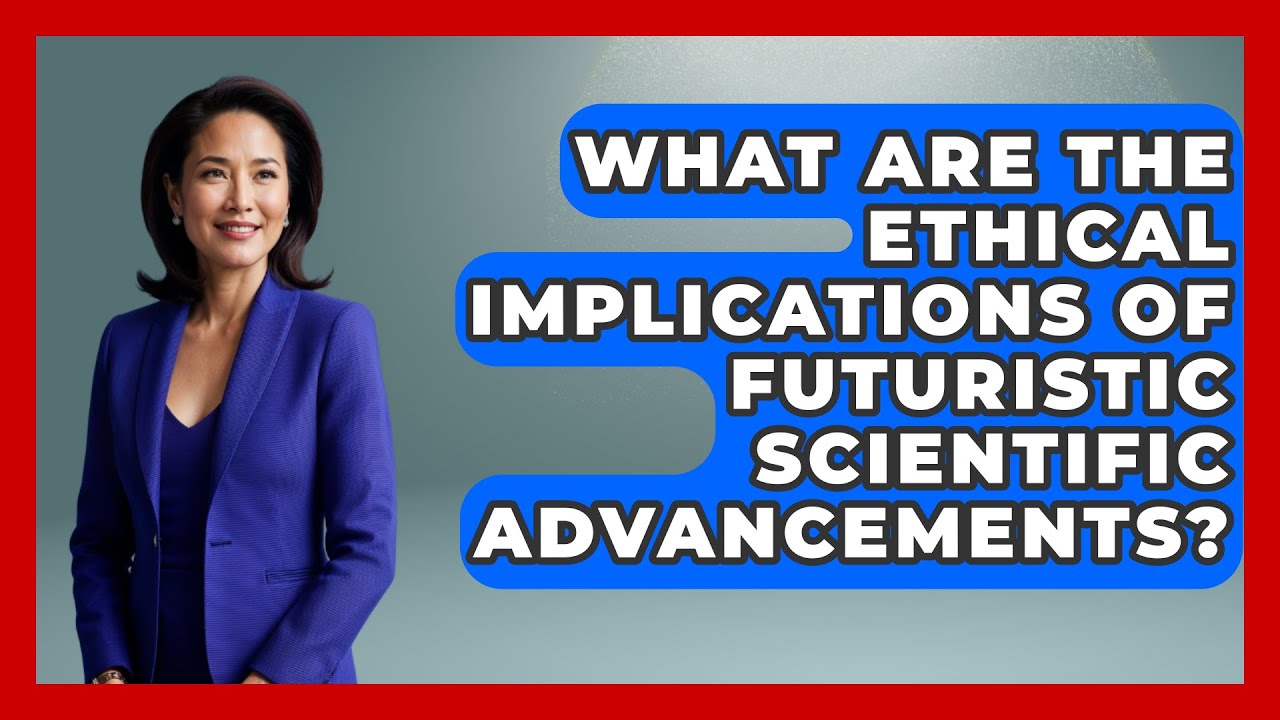
Image taken from the YouTube channel Strange Science HQ , from the video titled What Are the Ethical Implications of Futuristic Scientific Advancements? | Strange Science HQ .
Navigating the Ethical Minefield of AI
Artificial intelligence is no longer a futuristic fantasy. It is a pervasive force reshaping our world in profound ways. From powering search engines and recommending products to diagnosing diseases and driving cars, AI's influence is rapidly expanding.
Its capabilities are becoming increasingly sophisticated. This necessitates a critical examination of the ethical challenges it presents.
The Pervasive Influence of AI
AI's impact can be seen across numerous sectors.
- Healthcare utilizes AI for faster, more accurate diagnoses.
- Finance employs AI to detect fraud and personalize financial services.
- Transportation is being revolutionized by self-driving vehicles.
- Entertainment uses AI to curate content and create immersive experiences.
This rapid proliferation of AI demands a proactive and thoughtful approach to address the ethical dilemmas that arise.
Understanding AI: A Brief Overview
At its core, AI involves creating computer systems that can perform tasks that typically require human intelligence. These tasks include:
- Learning.
- Problem-solving.
- Decision-making.
- Pattern recognition.
AI systems achieve this through various techniques, including machine learning.
Machine learning algorithms allow computers to learn from data without explicit programming. This enables AI to adapt and improve its performance over time. However, this learning process also introduces ethical risks.
The Ethical Crossroads: A Looming Crisis
While AI holds immense potential to benefit society, its unbridled advancement poses significant ethical challenges. These challenges include:
- Bias: AI systems can perpetuate and amplify existing societal biases, leading to discriminatory outcomes.
- Accountability: Determining who is responsible when AI systems make mistakes or cause harm is a complex issue.
- Transparency: The "black box" nature of some AI models makes it difficult to understand how they arrive at decisions.
- Data Privacy: The extensive data required to train AI models raises concerns about the misuse of personal information.
Thesis: AI's vast potential is overshadowed by critical ethical concerns. Bias, accountability, transparency, and data privacy risks demand proactive solutions to ensure responsible AI development and deployment.
Addressing these ethical challenges is not merely an academic exercise. It is a fundamental imperative to ensure that AI serves humanity in a fair, equitable, and trustworthy manner. Failure to do so could have far-reaching and detrimental consequences.
Unveiling the Core Ethical Challenges of AI
The rapid advancement and integration of artificial intelligence into various aspects of society have brought to the forefront a complex web of ethical challenges. It is crucial to examine these core issues that threaten to undermine the potential benefits of AI. This section will delve into the critical ethical dilemmas that plague AI development and deployment. Concrete examples will be used to illustrate these problems and explore their potentially far-reaching consequences.
Bias in AI: Perpetuating Inequality
AI systems, while seemingly objective, can inadvertently perpetuate and even amplify existing societal biases. This occurs when biased data is used to train these systems. It also occurs when flawed algorithms are employed, or when human biases are embedded within the design of the AI.
Sources of AI Bias
Bias in AI arises from multiple sources. Biased data reflects existing societal inequalities, leading AI to learn and reproduce these biases. For example, if a hiring algorithm is trained on historical data that favors male candidates, it will likely perpetuate this bias in its future recommendations. Flawed algorithms, even those designed with good intentions, can unintentionally discriminate against certain groups. Finally, human biases, whether conscious or unconscious, can influence the design and development of AI systems, further contributing to biased outcomes.
Real-World Impacts of AI Bias
The impact of AI bias is felt across various sectors. In hiring, AI-powered tools can discriminate against qualified candidates from underrepresented groups. Loan applications can be unfairly denied based on biased credit scoring algorithms. In criminal justice, biased AI systems can lead to disproportionately harsh sentences for individuals from certain racial or ethnic backgrounds. These examples highlight the urgent need for fairness and equity in AI development and deployment.
The Imperative of Fairness and Equity
Addressing bias in AI requires a multi-faceted approach. This includes careful data collection and pre-processing to mitigate existing biases. Algorithmic fairness techniques can be employed to ensure that AI systems do not discriminate against any particular group. It also requires ongoing monitoring and evaluation to identify and correct any biases that may arise. Fairness and equity must be at the forefront of AI development and deployment.
Data Privacy: Protecting Personal Information in the Age of AI
AI models require vast amounts of data to train effectively. This reliance on data raises significant concerns about the privacy of personal information. The collection, storage, and use of personal data by AI systems pose serious risks. These risks include data breaches, identity theft, and the misuse of data for surveillance or discriminatory purposes.
The Extensive Data Requirements of AI
Training AI models demands extensive datasets, often containing sensitive personal information. This data can include demographic data, financial records, health information, and browsing history. The sheer volume of data required creates a significant vulnerability for privacy breaches.
Data Privacy Regulations and Compliance
To address these concerns, various data privacy regulations have been enacted. The General Data Protection Regulation (GDPR) in Europe and the California Consumer Privacy Act (CCPA) are examples. These regulations aim to protect individuals' rights to privacy and control over their personal data. However, compliance with these regulations can be challenging in an AI-driven environment, particularly when data is processed across multiple jurisdictions.
Privacy-Preserving Techniques
To mitigate privacy risks, researchers have developed various privacy-preserving techniques. Differential privacy adds noise to data to protect the privacy of individual data points. Federated learning allows AI models to be trained on decentralized data sources without directly accessing the raw data. These techniques offer promising avenues for developing AI systems that respect individuals' privacy.
Accountability and Responsibility: Who's to Blame When AI Errs?
One of the most perplexing ethical challenges in AI is determining accountability when these systems make mistakes or cause harm. When a self-driving car causes an accident, who is responsible? Is it the manufacturer, the programmer, or the owner of the vehicle? This lack of clear accountability poses a serious obstacle to the responsible development and deployment of AI.
The Difficulty of Assigning Responsibility
Assigning responsibility in AI-related incidents is complicated by the complex nature of these systems. AI models are often trained on vast datasets and involve intricate algorithms. This makes it difficult to trace the source of errors or biases. Moreover, AI systems can evolve and adapt over time, making it challenging to predict their behavior.
The Need for Clear Lines of Accountability
Establishing clear lines of accountability is crucial for building trust in AI. This requires defining the roles and responsibilities of all stakeholders involved in the AI lifecycle, including developers, deployers, and users. It also requires developing mechanisms for investigating and addressing AI-related incidents.
Approaches to AI Accountability
Several approaches can be employed to enhance AI accountability. Algorithmic audits can be used to assess the fairness, accuracy, and transparency of AI systems. Ethical impact assessments can help identify and mitigate potential ethical risks before AI systems are deployed. These proactive measures can promote responsible AI development.
Transparency and Explainability: Peering into the AI Black Box
Many AI models, particularly deep learning models, are often referred to as "black boxes". This is because their decision-making processes are opaque and difficult to understand. This lack of transparency raises concerns about bias, fairness, and accountability.
The "Black Box" Nature of AI
The "black box" nature of AI models makes it challenging to identify and correct errors or biases. It can also undermine trust in AI systems, particularly when their decisions have significant consequences. Without transparency, it is difficult to ensure that AI systems are operating fairly and ethically.
The Importance of Transparency and Explainable AI (XAI)
Transparency and explainability are essential for building trust and ensuring fairness in AI. Explainable AI (XAI) aims to develop techniques that make AI decision-making processes more transparent and understandable. XAI can help users understand why an AI system made a particular decision, enabling them to identify and correct any biases or errors.
Techniques for Enhancing AI Explainability
Various techniques can be used to enhance AI explainability. SHAP (SHapley Additive exPlanations) values provide a way to quantify the contribution of each feature to a model's prediction. LIME (Local Interpretable Model-agnostic Explanations) provides local explanations for individual predictions by approximating the model with a simpler, interpretable model in the vicinity of the prediction. These techniques can help users understand and trust AI systems.
Societal Implications: AI's Broader Impact
Having addressed the core ethical dilemmas inherent in AI development, it is crucial to broaden our lens and consider the far-reaching societal consequences that AI technologies are already beginning to unleash. From the transformation of the labor market to the pervasive expansion of surveillance capabilities, and even to the prospect of autonomous weaponry, AI presents a complex tapestry of challenges that demand careful scrutiny.
Job Displacement: AI and the Future of Work
One of the most pressing concerns surrounding AI is its potential to automate jobs and displace human workers. As AI-powered systems become increasingly capable of performing tasks previously requiring human intelligence, the labor market faces a significant upheaval.
The potential for widespread job displacement is not merely a theoretical concern; it is a rapidly unfolding reality.
Industries ranging from manufacturing and transportation to customer service and even white-collar professions are already experiencing the impact of AI-driven automation. While some argue that AI will create new jobs to offset these losses, the nature and distribution of these new opportunities remain uncertain.
Mitigating Job Displacement
To navigate this transition, proactive strategies are essential. Retraining programs designed to equip workers with the skills needed for the AI-driven economy are crucial. These programs should focus on areas such as AI development, data science, and roles that require uniquely human skills like creativity, critical thinking, and emotional intelligence.
Furthermore, exploring alternative economic models, such as universal basic income (UBI), may be necessary to ensure a basic standard of living for those displaced by automation. UBI could provide a safety net, allowing individuals to pursue education, entrepreneurship, or other activities that contribute to society.
Surveillance and Control: AI and the Erosion of Privacy
The use of AI for surveillance purposes raises profound ethical questions about privacy, civil liberties, and the potential for abuse of power. AI-powered surveillance systems, including facial recognition technology, are becoming increasingly sophisticated and pervasive, enabling governments and corporations to monitor individuals' activities on an unprecedented scale.
This raises concerns about the potential for mass surveillance, chilling effects on free speech and assembly, and the erosion of individual autonomy.
Ethical Implications of Facial Recognition Technology
Facial recognition technology, in particular, poses significant risks. While it can be used for legitimate purposes, such as identifying criminals or finding missing persons, it also has the potential to be misused for discriminatory or oppressive purposes. The technology's accuracy is not uniform across all demographics, with studies showing that it is often less accurate in identifying individuals from marginalized groups. This can lead to misidentification and wrongful accusations.
Protecting Civil Liberties
To prevent AI-powered surveillance from becoming a tool of oppression, robust safeguards are needed. These include strict regulations on the collection, storage, and use of personal data, as well as transparency requirements for surveillance systems. Independent oversight bodies are essential to ensure that these regulations are enforced and that civil liberties are protected.
Autonomous Weapons: The Ethics of Lethal AI
The development of autonomous weapons systems (AWS), also known as "killer robots," presents one of the most daunting ethical challenges of our time. These are weapons systems that can select and engage targets without human intervention, raising fundamental questions about accountability, responsibility, and the future of warfare.
The prospect of machines making life-or-death decisions without human oversight is deeply troubling.
Unintended Consequences and Escalation of Conflict
One of the primary concerns is the potential for unintended consequences. AWS could malfunction, make errors in target identification, or be vulnerable to hacking, leading to civilian casualties or unintended escalation of conflict.
Furthermore, the deployment of AWS could lower the threshold for war, as nations may be more willing to engage in conflict if they do not risk the lives of their own soldiers.
Arguments For and Against Autonomous Weapons
Proponents of AWS argue that they could potentially reduce human error in warfare and minimize civilian casualties. They also argue that AWS could be more effective than human soldiers in certain situations, such as operating in hazardous environments.
However, opponents argue that AWS are inherently unethical because they remove human judgment from the decision to take a life. They also argue that AWS could lead to an arms race, destabilizing international security. A global ban on the development and deployment of AWS is the position of many.
Facial Recognition Technology: A Double-Edged Sword
Facial recognition technology, while offering potential benefits in areas like security and convenience, presents significant privacy risks and raises concerns about bias and accuracy, particularly for marginalized groups.
Its ability to identify and track individuals without their knowledge or consent creates opportunities for misuse and abuse.
Bias and Accuracy
Bias in facial recognition algorithms is a significant problem. If the algorithms are trained on biased datasets, they will likely perpetuate and amplify these biases in their predictions. This can lead to misidentification and discrimination, particularly for people of color, women, and transgender individuals.
For instance, studies have shown that facial recognition systems are often less accurate in identifying people of color than white people, leading to a higher risk of false positives and wrongful accusations.
The Importance of Fairness and Accuracy
To mitigate these risks, it is essential to ensure that facial recognition algorithms are fair, accurate, and transparent. This requires careful data curation, algorithm design, and ongoing monitoring. Independent audits and testing are needed to identify and address biases in the systems.
Having illuminated the ethical quandaries that AI development inevitably presents, the vital question now becomes: how do we navigate this complex terrain responsibly? The path forward requires a multi-faceted approach, encompassing both robust regulatory frameworks and a fundamental shift towards proactive ethical development practices. These solutions aim not to stifle innovation, but to guide it towards a future where AI benefits humanity as a whole.
Addressing the Ethical Minefield: Solutions and Regulations
The EU AI Act: A Regulatory Blueprint?
The European Union, recognizing the profound implications of artificial intelligence, has taken a pioneering step with the development of the EU AI Act. This landmark legislation represents a comprehensive attempt to regulate AI development and deployment, setting a potential precedent for other nations and regions worldwide.
Key Provisions and Potential Impact
At its core, the EU AI Act adopts a risk-based approach, categorizing AI systems based on their potential to cause harm. Systems deemed to pose an unacceptable risk, such as those used for social scoring or subliminal manipulation, are outright banned.
High-risk AI systems, which include applications in areas like healthcare, law enforcement, and critical infrastructure, are subject to stringent requirements. These include:
-
Mandatory conformity assessments: Ensuring compliance with safety and ethical standards.
-
Data governance requirements: Addressing bias and ensuring data quality and integrity.
-
Transparency obligations: Providing clear information about the system's capabilities and limitations.
-
Human oversight mechanisms: Maintaining human control and accountability.
The impact of the EU AI Act is expected to be far-reaching. It will likely force companies developing and deploying AI systems in the EU to adhere to higher ethical and safety standards. This could lead to increased development costs and longer time-to-market.
However, it could also foster greater trust in AI systems and create a competitive advantage for companies that prioritize ethical AI development. The Act's influence may extend beyond the EU, as businesses operating globally may choose to adopt its standards to ensure compliance across all markets.
Risk-Based Regulation and Ethical AI
The EU AI Act's emphasis on risk-based regulation is a crucial element in promoting ethical AI. By focusing on the potential harms associated with different AI applications, the Act provides a targeted and proportionate approach to regulation. This avoids stifling innovation in low-risk areas while ensuring adequate safeguards are in place for high-risk applications.
The Act's provisions are designed to address some of the most pressing ethical concerns surrounding AI. The data governance requirements aim to mitigate bias in AI systems by ensuring that training data is representative and free from discriminatory patterns.
Transparency obligations promote accountability by making it easier to understand how AI systems make decisions. Human oversight mechanisms ensure that human beings retain ultimate control over AI systems, preventing them from operating autonomously in ways that could cause harm.
Fostering Ethical AI Development: A Proactive Approach
While regulation is essential, it is not sufficient to ensure the ethical development and deployment of AI. A truly ethical AI ecosystem requires a proactive approach that integrates ethical considerations into every phase of the AI lifecycle. This includes:
Embedding Ethics Throughout the AI Lifecycle
Ethical considerations should be integrated into every stage of AI development, from data collection and model design to deployment and monitoring. This requires a shift in mindset, where ethical principles are not treated as an afterthought but as a fundamental design constraint.
Data scientists and AI engineers need to be trained to identify and mitigate potential biases in data and algorithms.
Organizations need to establish clear ethical guidelines and governance structures to ensure that AI systems are developed and used responsibly.
The Role of Education and Training
Education and training are vital for promoting ethical AI practices. Data scientists, AI engineers, and policymakers need to be equipped with the knowledge and skills necessary to understand the ethical implications of AI and to develop and implement ethical AI solutions.
This includes training in areas such as:
-
Fairness and bias mitigation: Techniques for identifying and reducing bias in AI systems.
-
Data privacy and security: Methods for protecting personal information in AI applications.
-
Explainable AI (XAI): Approaches for making AI systems more transparent and understandable.
-
Ethical frameworks and principles: Guiding ethical decision-making in AI development and deployment.
Collaboration: A Cornerstone of Ethical AI
Developing and implementing ethical AI guidelines requires collaboration among researchers, policymakers, and industry. Researchers can contribute their expertise in AI ethics and fairness to develop practical solutions for mitigating bias and promoting transparency.
Policymakers can create regulatory frameworks that promote ethical AI development while fostering innovation. Industry can play a vital role by adopting ethical AI practices and sharing best practices with the broader community.
Through collaboration, we can create a future where AI is developed and used in a way that benefits all of humanity. This collaborative spirit is essential to navigating the complexities and ensuring a future where AI serves as a force for good.
Video: AI's Ethical Minefield: What Are the Implications?
AI's Ethical Minefield: Frequently Asked Questions
Navigating the complexities of AI ethics can be challenging. Here are some frequently asked questions to help clarify the key issues.
What are the main areas of ethical concern in AI development?
Key ethical implications in AI revolve around bias and fairness, accountability and transparency, privacy and security, and the potential for job displacement. Addressing these areas ensures responsible AI development and deployment.
How can AI bias lead to unfair outcomes?
AI algorithms are trained on data, and if that data reflects existing societal biases, the AI will perpetuate and even amplify those biases. This can lead to unfair or discriminatory outcomes in areas like loan applications, hiring processes, and even criminal justice. These are significant ethical implications of AI.
Who is responsible when an AI system makes a mistake?
Determining responsibility for AI errors is complex. Is it the programmer, the data provider, or the user? Establishing clear lines of accountability is crucial. Ignoring this issue has serious ethical implications.
What safeguards can be implemented to mitigate ethical risks in AI?
Implementing rigorous testing for bias, establishing clear ethical guidelines, ensuring transparency in AI decision-making processes, and prioritizing human oversight are essential steps. These strategies help minimize the ethical implications and promote responsible AI innovation.